The Difference Between Theory and Theorem and What It Tells Us About Data ‘Science’
It Provides Yet Another Argument Against Data ‘Science’ as Science and Against Its’ Practitioners as Scientists
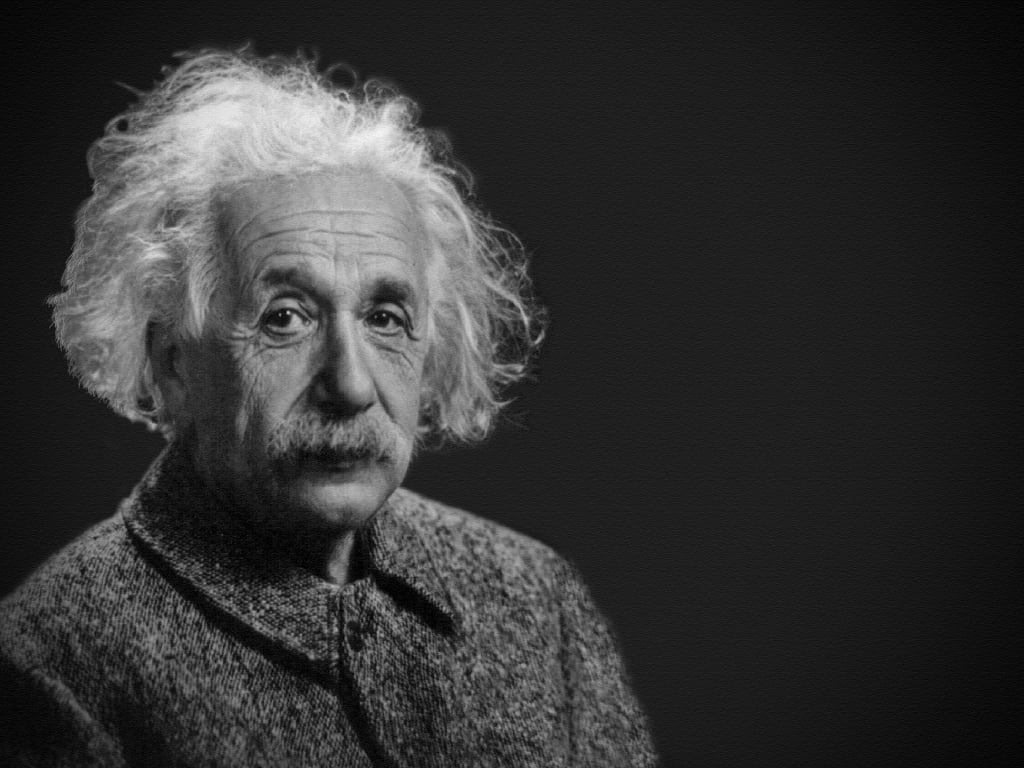
Theory vs. theorem
You might be asking yourself how I could possibly turn an article like this (the above linked) into yet another argument against data ‘science’ as science. It hinges on a critical, and sometimes underappreciated, difference between a theorem and a theory. In science, and as actual scientists, we hypothesize and if we are lucky generate enough data in support or against our hypotheses to develop theories which will allow us to explain that data. A theory is essentially just that, a set of ideas used to explain why something is (believed to be) true (if these ideas lack data to back support them they are only hypotheses). In contrast a theorem is a result that can be proven to be true from a set of axioms. The term is used most often in mathematics where the axioms are those of mathematical logic and the systems in question. A different and I think better way to put this is to say that a theory is an explanation that is (at least in principle) verifiable, while a theorem is an explanation that is/must be demonstrable. In this case verifiable means that one can show that there is evidence for it (empirical) while demonstrable means that you can do it again to show people the evidence, and that they can do it too (deductive).
Science and data "science"
Mathematics and mathematical logic make use of theorems, and are deductive, and they are not science. They are powerful tools of science to be sure, but they are not science. Yet for some reason a different (primarily deductive) tool of science and business and many other professions, data analytics, is said to be a science when the field itself uses primarily/exclusively mathematical and statistical tools to analyze existing data sets for various reasons. The data ‘scientist’ has no hand in creating the conditions that generate the data to be analyzed (i.e. designing or setting up the experiment), and in fact has no formal or informal training in the tools needed to so [e.g. design of experiments (DOE), basics of notebooking/recording/publishing, etc.]. The data ‘scientist’ does not hypothesize about a problem, design an experiment (either practical or theoretical), execute that experiment, analyze the results, and then draw conclusions based on those results to hypothesize further. At most they do two of those things and usually only one or none. From what I can tell most are not even aware of what each of those steps I just described are or how to apply them in any case. They are just not needed to do data ‘science’ as it is practiced today.
You may say that what I am describing only applies to research science and that not all science is research. Yes, you may say that, but all scientists, be they researchers or otherwise, are trained in the mechanics of doing research, the logistics of research, or its cadence. They all know how to do research even if they do not do it everyday. Moreover, many of the principles of research science apply to the work they do actually do.
Data science does not train its practitioners in these principles because they are not needed. When one is doing math one does not need to know where the numbers come from only that they exist and the rules for working with them. Similarly when one is doing data ‘science’ the origin/source of the data doesn’t really matter and almost never does the data ‘scientist’ have any hand in (how/why/what/when/where) said data is collected. They do not ‘design the experiment’ and do not have the tools or training to do so. They do not worry about recording their methods and results in ways that other experts in the field can replicate because their methods are already fully described and deductive, and by definition must be replicatable (demonstrable as I discussed above).
Not a value judgement
As I always try to emphasize whenever I discuss this topic, to say data science is not science is no value judgement as to its relative worth or merit. It is not to suggest that it is inferior or superior to actual science, only to show that it is different, it is not science. Clearly it is an extremely valuable and powerful tool. It’s practitioners are some of the brightest and sharpest and most clever people on the planet. That their work is highly valued is evidenced by the sky high salaries they are paid (especially when compared to actual scientists). To me as an actual working scientist it is puzzling to understand why data analytics professionals want so badly to be scientists. They have the high salaries and perks and flashy silicon valley jobs, all we have is lab coats, low pay, and some of the most low key, low visibility jobs you will find in any profession.
About the Creator
Everyday Junglist
Practicing mage of the natural sciences (Ph.D. micro/mol bio), Thought middle manager, Everyday Junglist, Boulderer, Cat lover, No tie shoelace user, Humorist, Argan oil aficionado. Occasional LinkedIn & Facebook user
Comments
There are no comments for this story
Be the first to respond and start the conversation.