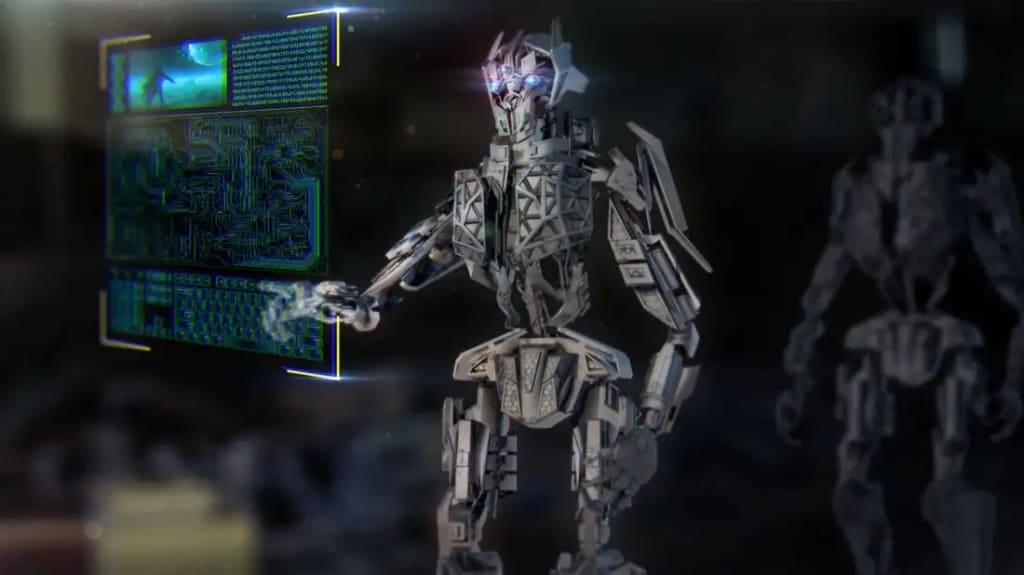
Ever-present artificial intelligence
We no longer have time for futuristic visions and idle debates like: “artificial intelligence — opportunity or threat?”. Instead of speculating about what this technology will “do to us” in the future, we can look at what we ourselves have done with it, looking for savings, convenience or answers to questions that have proven difficult for humans.
In the last decade, this technology has become firmly established in our smart devices, apps to measure everything, advertising, and anywhere else where it comes into play to influence human behavior: from assessing creditworthiness to managing public transportation and evaluating who is entitled to social benefits.
There’s no escaping the fact that decisions made or supported by artificial intelligence have a real impact on lives. At this stage — rulemaking to respond to the risks and secure the rights of people when they come into contact with such systems — the most important thing is to bring to the surface and subject to public scrutiny those decisions that on the surface seem technical but actually have both moral and political dimensions.
Does AI have to be a black box?
The discussion of accountability for the effects of AI-supported systems is greatly hampered by the persistent myth of the black box. In the media, such systems are most often portrayed as “magic boxes” that mere mortals can neither open nor comprehend (if they did, however). At the same time, we hear predictions that AI will decide about our lives: employment, treatment, credit. This is a real, and yet out of social control, power that we rightly fear.
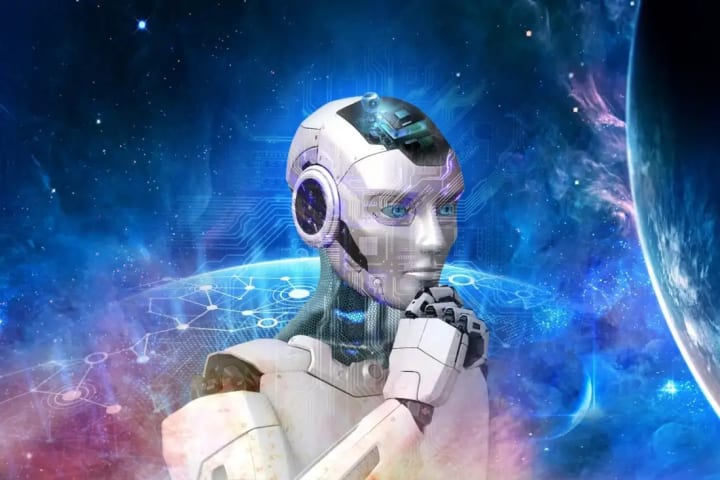
Especially since we have learned from high-profile examples — such as the U.S. COMPAS system that supports judges’ decisions — that AI makes tragic mistakes and, like society as a whole, can be biased. This crisis of trust could spill over very broadly into any application of data analytics to solve social problems.
But AI-based systems don’t have to be designed on a “black box” basis — in a way that doesn’t allow us to understand the final outcome or reconstruct the factors that influenced it. Various classes and types of models are available, including some that are as “openable” as possible, and even illustrate their operation in graphs that humanists can understand.
“Traditional” statistical models have been used for decades: in the social and life sciences, to predict trends in the stock market, and in medical research. Over that time, they have evolved so much, and we have gained so much data to train them, that they will perform better than experimental neural networks in many applications. That’s the advice designers of AI-based systems will also find in guidance that the UK’s Information Commissioner’s Office (ICO) has prepared in collaboration with the renowned Alan Turing Institute.
The guidance prepared by the British also leaves no doubt about the fact that it is not necessary to understand exactly how the model works (e.g. this mysterious neural network) to know what effect it has been optimized for. The point is what task has been set to the artificial intelligence and what effect it is supposed to have. This decision can always be extracted from the guts of the system and can always be discussed. This is the political — not technical — dimension of designing systems based on artificial intelligence.
Will AI predict our future?
Princeton computer science professor Arvind Narayanan, known for debunking AI systems that promise something that cannot be done, identifies three primary goals of artificial intelligence: it can support our perception, automate our judgment, and predict social outcomes. In his view, AI is getting better at the former, as it is employed to detect objectively existing patterns (e.g., classifying objects in photographs, detecting lesions in X-rays, translating speech into text).
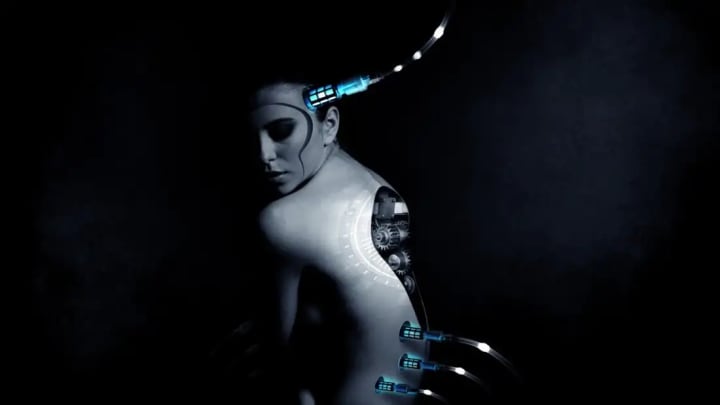
Much more difficult and controversial is the task of automating evaluation (e.g., detecting instances of hate speech online, distinguishing a sick person from a healthy one, tailoring content recommendations to a reader profile, etc.). Primarily because there is no unambiguously correct answer that the system can learn. Humans are also wrong in these cases, and context can matter gigantically. According to Narayanan, these systems will never be perfect, and it is for these systems that we need legal guarantees to protect people from wrong decisions.
Finally, the third category — systems that are designed to predict the future. It involves the greatest risk and, according to Narayanan, defies common sense. After all, we know that it is impossible to predict how a particular person will behave in the future. And yet we still try to do so — “thanks” to artificial intelligence. There is a fundamental difference between using AI to detect patterns and regularities that objectively exist and can be mathematically described, and employing it to look for patterns and regularities where they do not exist or are irregular.
There is also a fundamental difference between predicting a trend and trying to guess what a particular person will do. It is possible to predict an increase in the number of cars on the road or flu in the fall. We have representative data and can ask about an objectively existing problem. In the same way, however, we cannot predict who will commit a crime, to whom it is “profitable” to give social assistance, or who is worth hiring. From looking at the statistical regularity we cannot draw conclusions about a particular person. This is why artificial intelligence systems that support decisions about a group of people (e.g., judges in criminal cases, welfare officers, HR managers in large corporations) are justifiably controversial.
AI with a human face
We don’t need to invent the unthinkable and write a regulation that covers the gigantic, heterogeneous, and constantly evolving field that is what we commonly call “artificial intelligence.” It can focus on the decisions made by the people who design and use such systems to affect other people’s lives. It’s up to them to decide what rules autonomous vehicles will follow, how content will be profiled for us on the Internet, how risk assessment will be conducted in financial services, or “optimized” public policies.
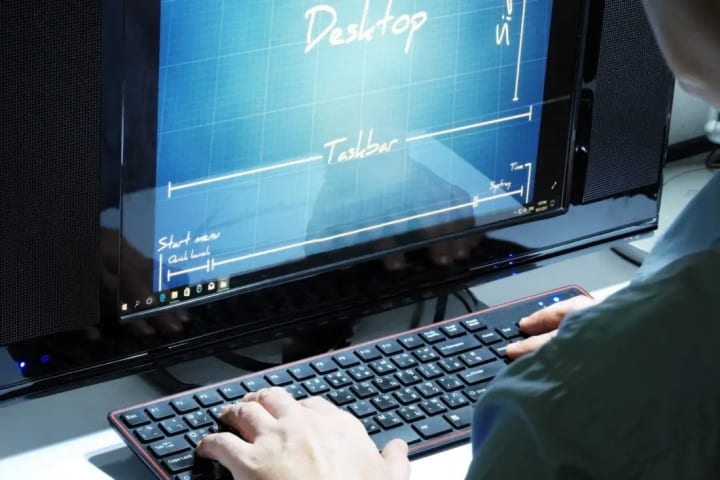
The point is not to get into regulating the proverbial Excel (which uses quite advanced mathematical functions), and at the same time not to overlook an area that — like personalized advertising — on the surface seems innocent, but in practice raises serious problems. And because we are talking about human decisions, of which there are many in the process of designing and calibrating artificial intelligence systems, we do not need to enter into expert arguments about whether the principles of statistical models can actually be explained. We can focus on what is human, intentional, and political. We can focus on the choices that can easily translate into who will gain and who will lose from the operation of a particular system.
A very good tool for such analysis is the system impact assessment, which is carried out in the early design phase, the so-called impact assessment. The idea is by no means new: it functions in the law-making process and in personal data protection. Similar methods can be applied to automated decision-making systems, which sometimes require the processing of personal data and sometimes do not. Algorithms resemble legal rules, only written in the language of mathematics. The effects of their impact on people can and must be assessed.
Mandatory impact assessment must become the rule. Of course, it is not only in public policy, but it is worth starting from this area to develop a gold standard. If the state takes on the implementation of artificial intelligence systems, it must have evidence that the specific problem it wants to solve can be described in the language of mathematical formulas. If it is that we don’t know something — such as how to diagnose a disease early — then data analytics is a good tool. But if the problem is that we don’t have the resources to treat all patients and we need to select “priority” cases, artificial intelligence will at best help buy time or mask a hole in the budget. In the long run, it’s a harmful policy that has to come back with a vengeance.
Comments
There are no comments for this story
Be the first to respond and start the conversation.