How Vector Databases Shape the Future of Artificial Intelligence
Do you know the vector databases role in shaping the future of AI? Dive in.
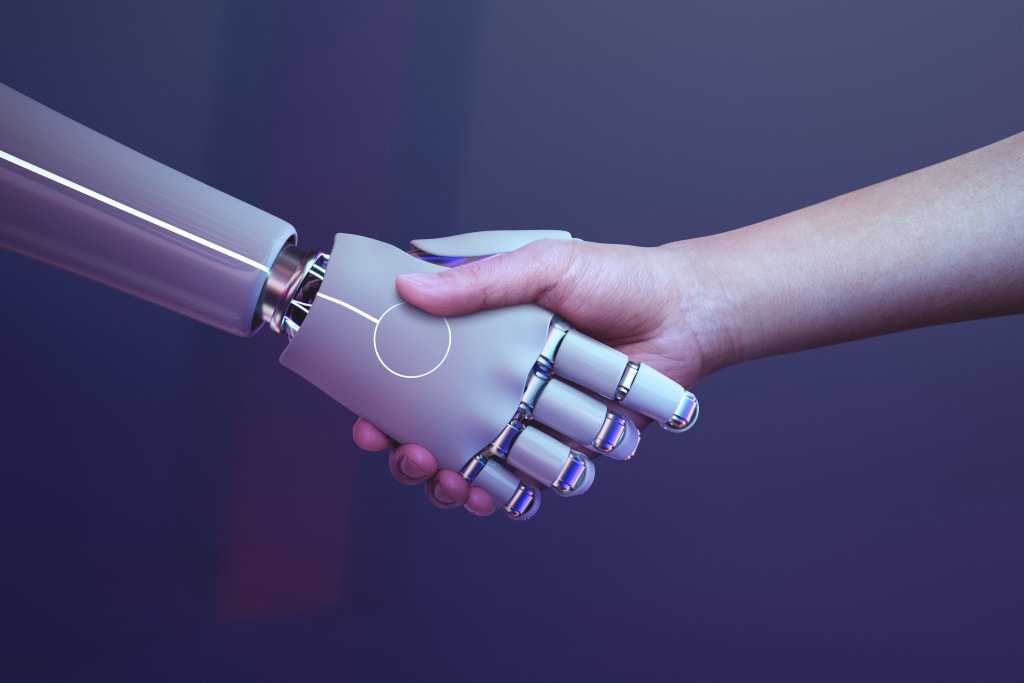
In today's tech landscape, Artificial Intelligence (AI) is voracious for data. With an increasing number of enterprise organizations adopting an AI-first strategy to enhance efficiency and gain a competitive edge, the establishment of what could be termed an 'AI factory' becomes paramount. This entails developing new generative AI functionalities and reinforcing the utilization of predictive and causal AI models, potentially industrializing decision-making processes within enterprises.
Enterprises leverage AI to sift through vast repositories of data, uncovering patterns, providing context, and automating processes to enhance the productivity of both knowledge workers and customer satisfaction. However, there exists a significant gap between merely accessing extensive datasets and blindly inputting them into AI algorithms versus strategically utilizing AI to anticipate market trends and meet customer demands.
To achieve the necessary performance levels for industrializing AI-driven decision-making, companies must first focus on establishing robust data services infrastructure. This infrastructure encompasses data ingestion capabilities, processing frequency, and proficiency in analytics and search functionalities, particularly across Large Language Models (LLMs). Traditional databases are often criticized for their limited computational abilities when handling temporal and unstructured data, which constitute the majority of datasets. Consequently, discussions around vector databases have gained prominence in recent months.
KX, a company specializing in high-performance time-series vector data management, stands out for its expertise in working with some of the most data-intensive applications across various sectors such as investment banking, life and health sciences, semiconductor development, telecommunications, and manufacturing.
How to Build an Infancy AI Factory
In the journey towards embracing the future, AI-first companies must evolve beyond traditional task automation and embrace a comprehensive enhancement of job processes. Ashok Reddy, CEO of KX, emphasizes the necessity of advanced technologies in achieving this transformation.
Reddy envisions not just augmenting individual tasks but revolutionizing entire job functions, empowering professionals to assume roles of greater strategic significance. This paradigm shift redefines the relationship between the human workforce and technological applications.
According to Reddy, realizing the concept of the AI factory for AI-first companies involves leveraging sophisticated pattern recognition technologies. This entails employing a range of AI tools such as Large Language Models (LLMs) and Retrieval Augmented Generation (RAG) to operationalize and industrialize decision-making processes across organizations.
An integrated approach fosters collaboration and eliminates siloed operations, fostering synergy across departments—an essential aspect of the AI-first business model. It's crucial to acknowledge the collaborative role of AI within this framework.
In an AI-first context, the objective is to complement rather than replace human intelligence. While AI excels in efficiency in pattern detection and data processing, it's human intuition and contextual insight that guide its application.
When will the Data Happen
If the concept of the AI factory holds validity, it necessitates the provision of tools and equipment to populate the shop floor. At this juncture, it becomes apparent that AI has the potential to enhance users' comprehension of how time influences their businesses and offer analytical insights for informed decision-making. The aim is to extract actionable knowledge from various data types, including text, video, audio, and images, thereby improving the relevance of data searches for AI-first companies.
Reddy and his team propose that empowered workers can leverage this knowledge to formulate long-term business strategies aligned with time-sensitive factors such as stock market fluctuations and customer purchasing patterns. For instance, analysts could delve into grain prices beyond mere market values, incorporating temporal analysis related to weather patterns, elections, export restrictions, or global conflicts.
"Through the application of time-oriented vector-based analytics, we significantly enhance the intelligence layer within AI-first companies, empowering professionals across diverse sectors like healthcare and law to broaden their capabilities," explains Reddy. "This approach serves as the foundation for advancing AI and LLMs, underscoring the importance of systems-level thinking in innovating workflows and workloads within the AI factory model."
About the Creator
Chandan Saxena
Chandan Saxena is a result-focused IT pro with 17+ years in cards and payment, specializing in card personalization, EMV, and ISO8583. Adept in the latest technology. A leader translating business needs into scalable solutions.
Comments
There are no comments for this story
Be the first to respond and start the conversation.