The Role of AI in Identifying Smurfing Operations in Money Laundering
Harnessing AI: Revolutionizing Smurfing Detection for Enhanced Financial Security and Regulatory Compliance Worldwide
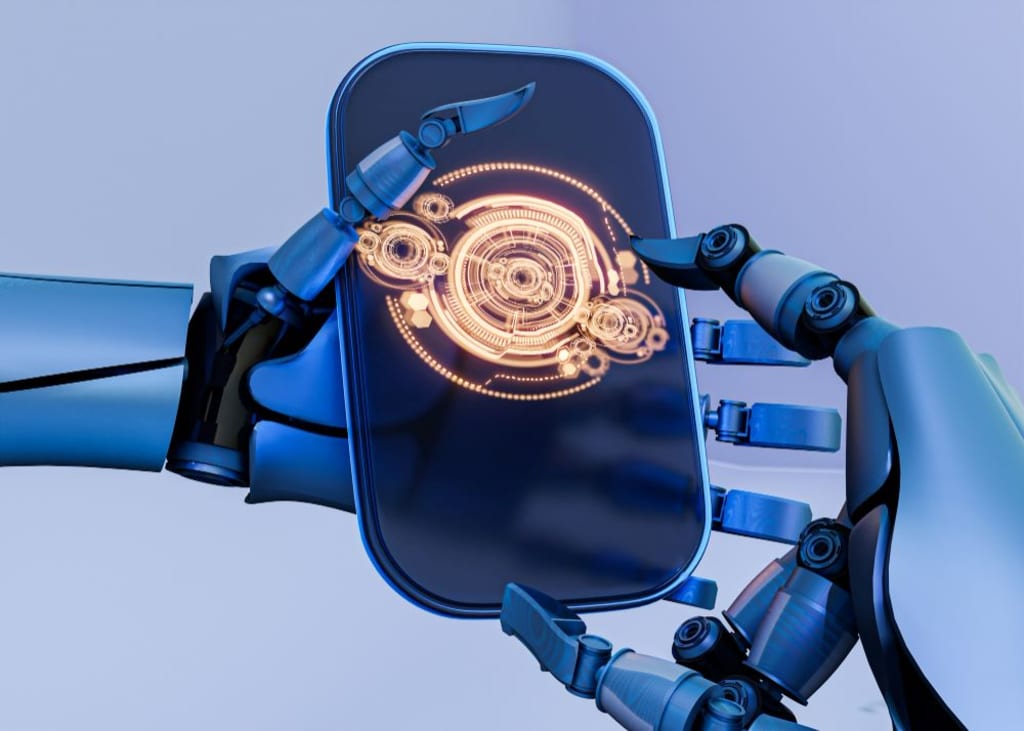
Smurfing is a money laundering technique used to avoid the detection of large financial transactions. This method involves breaking down large sums of money into smaller amounts, which are then deposited into various bank accounts to avoid triggering suspicious activity reports.
Given the complexity and sophistication of smurfing operations, traditional methods of detection often fall short. This is where Artificial Intelligence (AI) comes into play, offering enhanced capabilities to identify and prevent such activities.
Understanding Smurfing Operations
Smurfing, is characterized by its systematic and deliberate approach to splitting transactions. Perpetrators make numerous small deposits, typically below the reporting threshold, into various accounts.
This fragmentation makes it difficult for traditional monitoring systems to detect suspicious patterns. Recognizing these patterns requires analyzing large volumes of transaction data, which is where AI can significantly contribute.
Importance of Detecting Smurfing in the Placement Stage
Detection of smurfing is crucial in the placement stage of money laundering, where illicit funds are first introduced into the financial system.
The Role of AI in Detecting Smurfing
Introduction to AI and Machine Learning
Artificial Intelligence (AI) and Machine Learning (ML) involve the use of algorithms and statistical models to analyze and interpret complex data. In the context of anti-money laundering (AML), AI can process vast amounts of transaction data, identifying patterns and anomalies indicative of smurfing activities.
AI Techniques Used in Identifying Smurfing
Supervised Learning Models
Supervised learning involves training an AI model on labeled data, where the outcomes are known. This training enables the model to learn from past data and make predictions on new data.
Training Data and Labeling: Financial institutions provide historical transaction data, labeled as either normal or suspicious. The AI model uses this data to learn the characteristics of smurfing.
Algorithms and Their Application: Common algorithms include decision trees, support vector machines, and neural networks. These algorithms help in predicting whether a transaction is likely to be part of a smurfing operation.
Unsupervised Learning Models
Unsupervised learning models do not rely on labeled data. Instead, they identify anomalies and clusters within the data.
Anomaly Detection Techniques: These techniques identify transactions that deviate significantly from typical patterns. Anomalies often indicate potential smurfing activities.
Clustering Algorithms: Clustering groups similar transactions together, making it easier to spot unusual patterns that may indicate smurfing.
Neural Networks and Deep Learning
Neural networks, particularly deep learning models, are highly effective in identifying complex patterns in large datasets.
- Convolutional Neural Networks (CNNs): Though typically used in image processing, CNNs can be adapted for transactional data to identify intricate patterns.
- Recurrent Neural Networks (RNNs): RNNs are useful for sequence prediction, making them ideal for detecting sequences of transactions indicative of smurfing.
Implementation of AI in Financial Institutions
Integrating AI with Existing AML Systems
Financial institutions can integrate AI with their existing AML systems to enhance detection capabilities. This integration involves incorporating AI algorithms into transaction monitoring systems and training staff on their use.
Data Requirements and Management
Implementing AI requires extensive data management, including the collection, cleaning, and storage of transaction data. High-quality data is crucial for effective AI model training and operation.
AI Tools and Software Used in Detection
There are various AI tools and software solutions available for detecting smurfing, such NICE Actimize. These tools offer advanced analytics and real-time monitoring capabilities.
Training and Development for AML Professionals
AML professionals need training to effectively use AI tools. This training includes understanding how AI models work, interpreting their outputs, and responding to alerts generated by AI systems.
Case Studies and Real-World Applications
Case Study 1: Successful AI Implementation in a Major Bank
A major bank implemented an AI-driven AML system to combat smurfing. The bank integrated AI with its existing systems, resulting in a significant increase in the detection of suspicious activities. The AI system identified complex patterns and provided real-time alerts, enabling quicker responses.
Case Study 2: AI in an International Financial Institution
An international financial institution faced challenges with cross-border smurfing. By implementing AI, the institution improved its ability to detect and prevent smurfing activities. The AI system collaborated with regulatory bodies, enhancing compliance and reporting.
Case Study 3: Small and Medium-Sized Enterprises (SMEs)
SMEs often lack the resources for sophisticated AML systems. However, affordable AI solutions tailored for SMEs have proven effective. These solutions use cloud-based AI tools to monitor transactions, offering SMEs robust AML capabilities.
Challenges and Limitations of AI in Smurfing Detection
Data Quality and Availability
AI systems require high-quality data for effective operation. Inconsistent or incomplete data can hinder the performance of AI models.
False Positives and False Negatives
AI models can generate false positives (incorrectly flagged transactions) and false negatives (missed suspicious transactions). Balancing sensitivity and specificity is crucial to minimize these errors.
Complexity of AI Algorithms and Models
AI algorithms can be complex, requiring expertise to develop and maintain. Financial institutions need skilled personnel to manage these systems effectively.
Regulatory and Ethical Concerns
The use of AI in AML raises regulatory and ethical concerns, including data privacy and the potential for biased algorithms. Ensuring compliance with regulations and ethical standards is essential.
Adaptability to Evolving Money Laundering Techniques
Money laundering techniques continuously evolve. AI models must be regularly updated and retrained to adapt to new patterns and methods used by criminals.
Future Trends and Developments
Advances in AI and Machine Learning for AML
Ongoing advancements in AI and machine learning promise improved AML capabilities. Future developments include more sophisticated algorithms and enhanced predictive analytics.
Integration of AI with Blockchain Technology
Integrating AI with blockchain technology can provide greater transparency and security in financial transactions. Blockchain’s immutable ledger, combined with AI’s analytical power, offers a robust solution for AML.
The Role of AI in Enhancing Global AML Collaboration
AI can facilitate better collaboration between financial institutions and regulatory bodies worldwide. Sharing AI-generated insights can improve the global fight against money laundering.
Emerging AI Tools and Platforms
New AI tools and platforms are continuously emerging, offering advanced features for AML. These tools leverage cutting-edge technologies to enhance detection and prevention capabilities.
Predictive Analytics and Proactive AML Measures
Predictive analytics enable proactive AML measures, allowing institutions to anticipate and mitigate risks before they materialize. AI-driven predictive models can identify potential threats early.
Conclusion
The role of AI in identifying smurfing operations is increasingly vital in the fight against money laundering. AI's ability to analyze vast amounts of data, recognize patterns, and provide real-time alerts significantly enhances the detection and prevention of smurfing.
While there are challenges and limitations, the continuous advancements in AI technology and its integration with AML practices offer promising solutions.
As financial institutions and regulatory bodies adopt and refine AI-driven AML systems, the effectiveness of combating money laundering will continue to improve, ensuring greater financial security and compliance.
About the Creator
Enjoyed the story? Support the Creator.
Subscribe for free to receive all their stories in your feed. You could also pledge your support or give them a one-off tip, letting them know you appreciate their work.
Comments
There are no comments for this story
Be the first to respond and start the conversation.