Nine ways for hackers to launch attacks with machine learning
Hacker technology
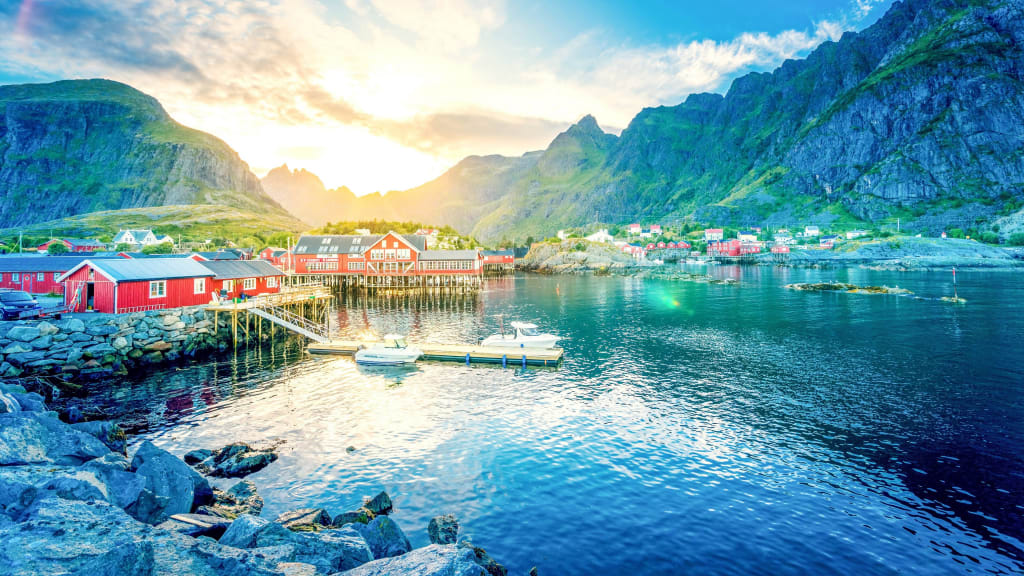
one
Spam, spam.
Fernando Montenegro, an analyst at Omdia, says defenders have been using machine learning to detect spam for decades, and spam detection is the best initial use case for machine learning.
However, if the spam filter leaks the detection method (or scoring criteria), attackers can use it to optimize attack behavior. For example, attackers will use legitimate tools to improve the success rate of attacks. "if you submit content frequently enough, the attacker can rebuild the model and then optimize the attack to bypass the model."
It's not just spam filters that are vulnerable. Montenegro points out that any security vendor that provides scores or other output information can be abused. Although not all vendors have this problem, if you are not careful, useful output will be leaked and attackers can use it for malicious purposes.
two
"better" phishing email
Attackers not only use machine learning security tools to test whether their messages can pass spam filters. Adam Malone, a partner at Ernst & Young, said attackers would also use machine learning to create phishing emails. Hackers peddle such services and tools on crime forums. Can be used to generate "better" phishing emails, including generating fake characters to promote fraudulent activity.
Machine learning allows attackers to customize phishing emails in a creative way, optimized to trigger participation and clicks. Not only do they optimize the text of email, artificial intelligence can also be used to generate realistic photos, social media materials and other materials to make communication as legal as possible.
three
Guess the password
Malone points out that criminals are also using machine learning to better guess passwords. "We have seen evidence of an increase in the frequency and success rate of password-based guessing engines." He said. Criminals are developing better dictionaries and cracking stolen hashes.
They also use machine learning to identify security controls, Malone said, "so they can reduce attempts and guess better passwords and increase their chances of successfully accessing the system."
four
Deep forgery
The most frightening use of artificial intelligence is a deep forgery tool that can generate fake video or audio. Being able to simulate someone's voice or face is very useful for social engineering attacks.
In fact, in the past few years, some high-profile cases of deep forgery have been made public, including one in which criminals cost the company millions of dollars by falsifying the voices of corporate executives.
More often, fraudsters use artificial intelligence technology to generate realistic photos, user profiles and phishing emails to make their information look more credible. This is big business. According to FBI, the fraud of commercial email leaks has caused more than $43 billion in losses since 2016. Last fall, there were media reports that a Hong Kong bank was tricked into transferring 35 million US dollars to a criminal gang because a bank official received a call from a company director he had talked to before, and he was familiar with the voice. so he authorized the transfer.
five
Bypass popular network security tools
Many of today's popular security tools are built into some form of artificial intelligence or machine learning. For example, antivirus tools are increasingly going beyond basic features to look for suspicious behavior. "anything available online, especially open source, can be exploited by the bad guys," said Kantarcioglu, a professor of computer science at the University of Texas. "attackers can use these tools to adjust their malware to evade detection. Artificial intelligence models have many blind spots, and you can evade detection by changing the characteristics of the attack, such as how many packets are being sent or which resources are being attacked. "
Attackers use not only artificial intelligence-driven security tools, but also other artificial intelligence technologies. For example, users often learn to find phishing emails by looking for syntax errors, while attackers begin to use artificial intelligence syntax checkers like Grammarly to improve their writing.
six
Reconnaissance
Machine learning can be used for reconnaissance so that attackers can view the target's traffic patterns, defense systems, and potential vulnerabilities. This is not an easy task, so ordinary cyber criminals are unlikely to participate in such activities. "you need some skills to use AI," Kantarcioglu said. "so I believe it is mainly high-level national hacker organizations that use AI at the moment."
However, if at some point the technology is commercialized and provided as a service through an underground criminal network, it may become more widely used. "this can also happen if a country hacker develops a specific toolkit that uses machine learning and publishes it to the criminal community," Kantarcioglu said. "but cybercriminals still need to understand the function and use of machine learning applications, and technical barriers still exist."
seven
Autonomous agent
If the enterprise detects the attack and disconnects the affected system, the malware may not be able to connect back to its command and control server to obtain instructions. "but attackers may develop an intelligent model that will persist for a long time, even if they can't control it directly," Kantarcioglus said. But for ordinary cyber crimes, I believe this will not be a priority. "
eight
Artificial intelligence poisoning
Attackers can deceive the machine learning model by providing interference (countermeasure) information. "attackers can manipulate training data sets," said Alexey Rubtsov, a senior researcher at the Global risk Institute. "for example, they deliberately create biases that lead to misjudgments in machine learning."
For example, a hijacked user account logs in to the system at 2 am every day to do harmless work, making the system think that there is nothing suspicious about working at 2 am, thus reducing the chance of being detected during an attack.
This is similar to the 2016 Microsoft chat robot Tay was "abetted" to become a racist. The same method can be used to mislead artificial intelligence systems, to determine a particular type of malware as a secure application, or to assume that a particular bot behavior is completely normal.
nine
Artificial intelligence fuzzy test
Legitimate software developers and penetration testers use fuzzy software to generate random sample input in an attempt to crash the application or discover vulnerabilities. Enhanced versions of the software use machine learning to generate input in a more centralized and structured manner, such as giving priority to text strings that are most likely to cause problems. This makes fuzzy testing tools more useful for enterprises, but also more lethal in the hands of attackers.
Conclusion: defense in depth is the most effective strategy against machine learning attacks.
Forrester's Mellen pointed out: by analyzing the various machine learning attack methods used by attackers, we find that basic network security measures such as patching, anti-phishing education and micro-segmentation are still very important, and enterprises need to set up multiple barriers and not rely too much on a single (AI) technical solution, so as to avoid the problem of attackers picking up your stone and hitting you in the foot.
download!
Comments
There are no comments for this story
Be the first to respond and start the conversation.