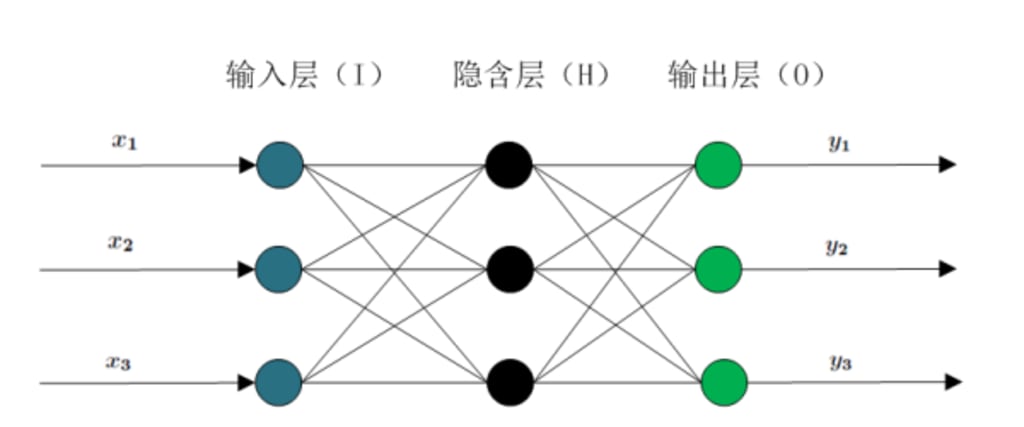
Introduction
Deep learning has emerged as a revolutionary technology in the field of artificial intelligence (AI) and has demonstrated remarkable capabilities in various applications, from image recognition to natural language processing. At the heart of deep learning are neural networks, which are inspired by the human brain's neural structure. In this essay, we will delve into the intricacies of neural networks, exploring how they learn and make decisions, and how these processes have the potential to reshape the future of AI from a professional perspective.
I. The Architecture of Neural Networks
Neural networks are composed of multiple layers of interconnected nodes, or neurons. The structure is designed to mimic the information processing capabilities of the human brain. Each neuron receives inputs, processes them, and produces an output that is passed to the next layer of neurons. The neural network's depth is a crucial factor that enables it to handle complex tasks, hence the name "deep learning."
II. The Learning Process: Backpropagation
The fundamental mechanism behind the learning ability of neural networks is known as backpropagation. During training, the neural network is fed with a large dataset, and its output is compared to the desired output. The difference between the predicted output and the actual output, known as the loss, is calculated.
Backpropagation works by propagating this loss backward through the network, adjusting the weights and biases of the neurons to minimize the error. The process is iterative and continues until the model reaches an acceptable level of accuracy on the training data.
III. Challenges in Training Deep Neural Networks
Training deep neural networks is not without challenges. The vanishing gradient problem occurs when the gradients become infinitesimally small, hindering the training process. Researchers have developed various techniques, such as batch normalization and skip connections, to address this issue.
Additionally, overfitting is a common problem in deep learning, where the model performs well on the training data but poorly on unseen data. Regularization methods, such as dropout and weight decay, help prevent overfitting and improve the generalization ability of the model.
IV. Decision Making in Neural Networks
Once trained, neural networks can make decisions by analyzing input data and producing an output. In classification tasks, the network assigns a probability score to each class and selects the class with the highest score as its decision. The decision-making process is based on the learned patterns and features from the training data.
For example, in image recognition, a deep neural network can identify objects in images based on the patterns it has learned from a vast dataset of labeled images during the training phase.
V. The Black Box Problem
While deep learning has demonstrated impressive capabilities, it is often referred to as a "black box" due to its opacity. The decision-making process in neural networks involves millions of interconnected parameters, making it challenging to interpret how the model arrives at a particular decision.
In some critical applications, such as medical diagnosis or autonomous vehicles, interpretability is crucial for building trust and understanding the model's decisions. Researchers are actively working on developing techniques to make neural networks more transparent and interpretable.
VI. Advances in Explainable AI (XAI)
Explainable AI (XAI) is a field dedicated to enhancing the interpretability of AI models, including neural networks. XAI methods aim to provide insights into the decision-making process of neural networks, making them more transparent and understandable.
One approach is to generate heatmaps that highlight the regions in an image that most influence the network's decision. These visualizations help identify the features and patterns that the model focuses on when making decisions.
VII. The Future of Neural Networks
Neural networks have already revolutionized various industries, including computer vision, natural language processing, and speech recognition. However, their potential is far from exhausted, and they are likely to play an increasingly significant role in shaping our future.
As computing power and data availability continue to increase, neural networks will become more sophisticated, allowing them to tackle even more complex and diverse tasks. Applications such as personalized medicine, climate prediction, and financial analysis stand to benefit from the advancements in deep learning.
Conclusion
Deep learning and its neural networks have proven to be a game-changer in the field of artificial intelligence. With the ability to learn from vast amounts of data and make decisions based on patterns and features, neural networks have shown impressive performance in various applications. While the "black box" problem remains a challenge, the research on explainable AI is paving the way to make neural networks more interpretable and trustworthy.
As we move forward, the continuous development of deep learning techniques will undoubtedly shape our professional landscape, providing solutions to previously unsolvable problems and unlocking the full potential of artificial intelligence. The future of neural networks is bright, and we can expect them to lead us into new frontiers of technological advancement and innovation.
Comments
There are no comments for this story
Be the first to respond and start the conversation.