Kemi Nelson Discusses the Potential of Synthetic Data in Data Science
Based in Dallas, Texas, Kemi Nelson is a driven businesswoman with years of experience in data and analytics executive in corporate contexts. In this article, Kemi Nelson reviews the potential of synthetic data in data science.
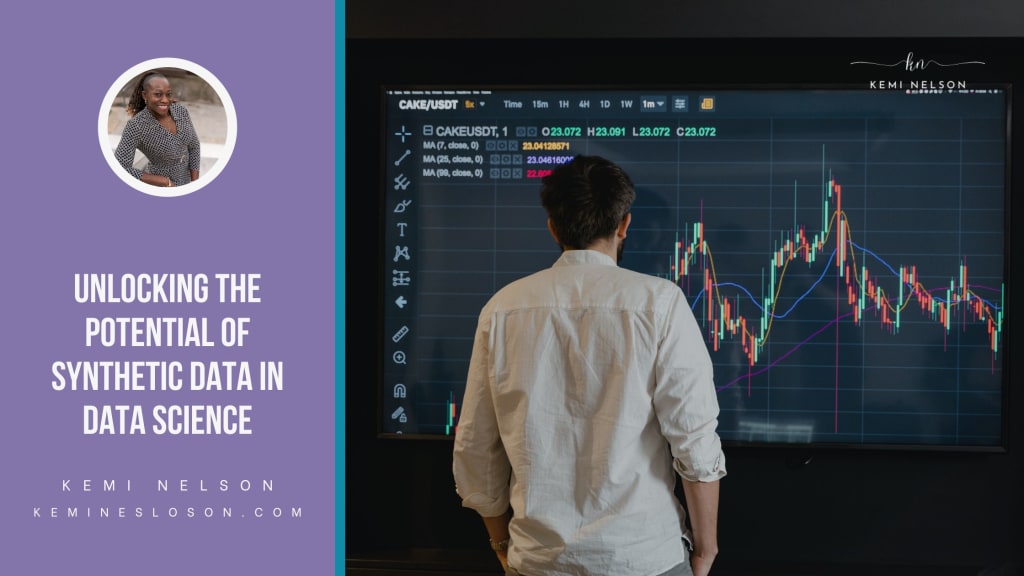
Artificial Intelligence (AI) and Machine Learning (ML) models have become increasingly popular in both the business and private sectors. They allow individuals and companies to generate images, text, audio, and more for little to no cost and cut down on the number of labor hours required to complete crucial workplace tasks. They can also be valuable assistants for creative and analytical minds alike, providing faster ways to complete menial or repetitive tasks. However, before AI/ML models can be utilized in these capacities, they must first be trained. Synthetic data provides a possible solution to the vast amount of information and time that must be dedicated to creating a functional AI model.
The Problems with Datasets
To effectively train an AI, it must be provided with a vast quantity of representative data about the task it needs to complete. If, for example, an AI needs to be able to identify a certain object in a photograph consistently, it will need to be shown as many photos of that object as possible. However, this can be a significant problem when creating an AI designed to do certain tasks. Many datasets that could provide valuable training for AIs for use in industries like finance contain private data that is protected by law, and unable to be used in the training. Certain data may be too cost-prohibitive to gather on a large scale, or may concern events that happen too rarely to gather a large sample size effectively. There are also instances in which the data has simply not been collected for a necessary dataset.
What is Synthetic Data?
Synthetic Data is data that is entirely fictional but is based on a real dataset. ML models have been created that can take an original dataset, analyze the relations between pieces of information, and create a new dataset that preserves that data’s statistical and relational significance but removes information specific to the real individuals providing the data. Synthetic Data programs can create entirely new datasets or remove identifiers from extant data.
How Can Synthetic Data Solve Common Problems with Real Datasets?
When Synthetic Data programs are used to create new information, that information can be disseminated much more freely than datasets containing personal identifiers. This helps businesses and data scientists overcome increasing privacy concerns from the public and stay within the boundaries of the law.
Synthetic Data can be used to train AI where there is insufficient real data to do so organically. Where data is unavailable due to privacy concerns or collection difficulty, such as in medical or financial fields, synthetic data extrapolated from consistent patterns in existing datasets may be able to provide enough information for the models to work accurately.
What are Some Future Use Cases for Synthetic Data?
In the future, synthetic data could be used to train medical AIs to detect and diagnose rare diseases. It also has the potential to mitigate some of the biases that AIs trained on real-world data exhibit. It has been demonstrated that minority populations are underrepresented in data used to train AIs, and therefore the algorithms can be biased against them. This is a major flaw in existing ML models; synthetic data may be the key to correcting it. Where data on minority individuals is missing, it could be possible for a sophisticated synthetic data program to produce new, accurate data extrapolated from what exists. This could then be used to retrain AI and correct their biases.
Are There Any Issues with Synthetic Data?
Like all new technologies, there are some problems that synthetic data still needs to work out.
It needs to be determined how synthetic data scientists can accurately measure the degree of privacy that synthetic data confers on a utilized dataset.
Synthetic data has also yet to be in common enough use for its accuracy to be proven to the necessary degree to train AI/ML models that would assist in high-risk fields like medicine.
When research on synthetic data progresses to the point that businesses and organizations can feel confident in its use, it will undoubtedly spark a revolution in data science. Synthetic data will allow for AI training that satisfies private citizens by protecting their privacy and benefits institutions by lowering costs.
About the Creator
Kemi Nelson
Kemi Nelson is a finance executive in Dallas, TX, with an impressive 15-year career in corporate leadership. She loves investing in people and has driven growth at every company she's worked with.
Comments
There are no comments for this story
Be the first to respond and start the conversation.