How can AI & Data Science startups effectively manage and analyze big data to extract valuable insights for decision-making?
Insights from Big Data: Strategies for AI & Data Science Start-ups!
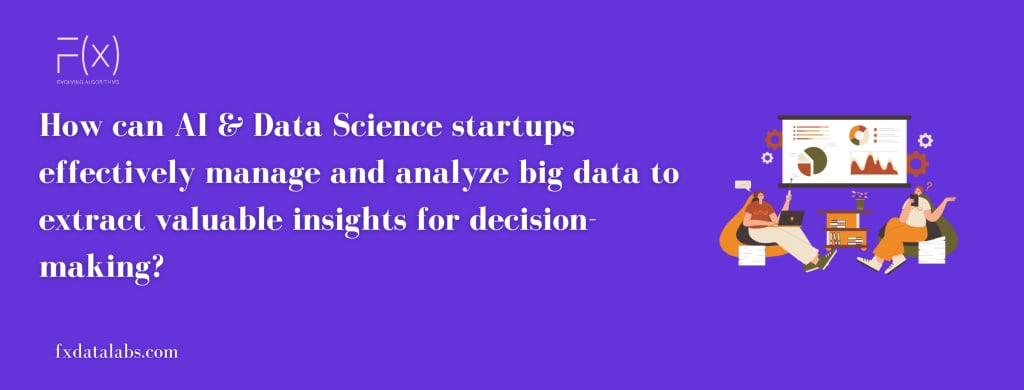
Introduction
In today's digital era, data has become the lifeblood of businesses, providing valuable insights that drive decision-making and strategy development.
For AI & Data Science start-ups, effectively managing and analyzing big data is essential for gaining a competitive edge, identifying market opportunities, and delivering innovative solutions.
However, the sheer volume, velocity, and variety of data pose significant challenges for start-ups looking to extract actionable insights.
In this article, we will explore how AI & Data Science start-ups can effectively manage and analyze big data to extract valuable insights for decision-making.
Understanding Big Data
Big data refers to large and complex datasets that exceed the processing capabilities of traditional data management systems. These datasets are characterized by the 3Vs: volume, velocity, and variety. Volume refers to the sheer size of data, velocity refers to the speed at which data is generated and processed, and variety refers to the different types and sources of data.
For AI & Data Science start-ups, big data sources may include structured data from databases, unstructured data from social media and web sources, and semi-structured data from IoT devices and sensors. However, the quality and relevance of data are equally important, as inaccurate or irrelevant data can lead to flawed insights and decisions.
Data Management Strategies
Effective data management is crucial for AI & Data Science start-ups to extract meaningful insights from big data. This includes data collection, aggregation, storage, preprocessing, and cleaning. Data collection techniques may involve web scraping, API integration, or manual data entry.
Once collected, data must be stored in scalable and secure infrastructure, such as cloud-based storage solutions or distributed file systems. Data preprocessing and cleaning involve removing duplicates, outliers, and errors, as well as transforming data into a usable format for analysis.
Big Data Analytics Tools and Techniques
A variety of tools and techniques are available for analyzing big data, ranging from traditional analytics frameworks to advanced machine learning algorithms.
Exploratory data analysis (EDA) techniques, such as data visualization and statistical analysis, help identify patterns, trends, and outliers in large datasets.
Machine learning algorithms, such as clustering, classification, and regression, enable predictive analytics and forecasting based on historical data.
Additionally, natural language processing (NLP) and deep learning techniques can extract insights from unstructured text and image data.
Extracting Insights from Big Data
The primary goal of big data analysis is to extract actionable insights that drive decision-making and strategy development. This involves identifying patterns, correlations, and causal relationships in large datasets.
Predictive analytics techniques, such as regression analysis and time series forecasting, enable AI & Data Science start-ups to anticipate future trends and outcomes based on historical data.
Data visualization tools, such as charts, graphs, and dashboards, provide intuitive representations of complex data, making it easier for stakeholders to interpret and act upon insights.
Challenges in Big Data Analysis
Despite its potential benefits, big data analysis poses several significant challenges for AI & Data Science start-ups. One of the primary challenges is scalability, as traditional data processing tools and techniques may struggle to handle the volume and velocity of big data.
Additionally, processing speed limitations can result in long processing times and delays in generating insights. Data privacy and security concerns are also paramount, especially when dealing with sensitive or proprietary information.
Ensuring compliance with data protection regulations and implementing robust security measures are essential for safeguarding data integrity and confidentiality.
Furthermore, the skills gap and talent shortage in data analytics pose a significant hurdle for start-ups, as recruiting and retaining qualified data scientists and engineers can be challenging.
Best Practices for Effective Data Analysis
To overcome these challenges, AI & Data Science start-ups should adopt best practices for effective data analysis. Firstly, it is essential to establish clear business objectives and goals for data analysis initiatives.
By aligning data analysis efforts with business priorities, start-ups can ensure that insights generated are relevant and actionable.
Collaboration with domain experts and data scientists is also crucial, as domain knowledge can provide valuable context and insights into data analysis processes.
Additionally, implementing agile and iterative approaches to data analysis allows start-ups to adapt quickly to changing requirements and refine their analytical models based on feedback and results.
Case Studies
Several successful AI & Data Science start-ups have leveraged big data analytics to drive innovation and achieve business success. For example, a healthcare start-up used predictive analytics and machine learning algorithms to analyze patient data and identify individuals at risk of developing chronic diseases.
By proactively targeting high-risk individuals with preventive interventions, the start-up was able to improve patient outcomes and reduce healthcare costs.
Similarly, an e-commerce start-up utilized data analytics to personalize product recommendations for customers based on their browsing and purchasing history, resulting in increased sales and customer satisfaction.
Future Trends and Innovations
Looking ahead, the future of big data analytics in AI & Data Science start-ups is bright, with several emerging technologies and trends shaping the landscape. One such trend is the increasing adoption of edge computing, which involves processing data closer to its source to reduce latency and bandwidth usage.
Another trend is the proliferation of augmented analytics platforms, which leverage machine learning and natural language processing to automate data analysis tasks and generate insights in real-time.
Additionally, advancements in data privacy-preserving technologies, such as federated learning and homomorphic encryption, are enabling start-ups to analyze sensitive data while protecting user privacy.
Conclusion
In conclusion, effective management and analysis of big data are critical for AI & Data Science start-ups to extract valuable insights for decision-making and strategy development.
By understanding the challenges, adopting best practices, and leveraging innovative technologies, start-ups can unlock the full potential of big data analytics and gain a competitive edge in the market.
For more insights into AI|ML and Data Science Development, please write to us at: [email protected]| F(x) Data Labs Pvt. Ltd.
#BigData #DataScience #AI #Analytics #DecisionMaking 📊🚀💡🔍
About the Creator
Fxdatalabs
Explore the epitome of technical excellence and innovation with F(x) Data Labs, India's leading data science company founded in 2015.
Comments
There are no comments for this story
Be the first to respond and start the conversation.