What Does Machine Learning Do for Data Science?
Machine Learning In Data Science
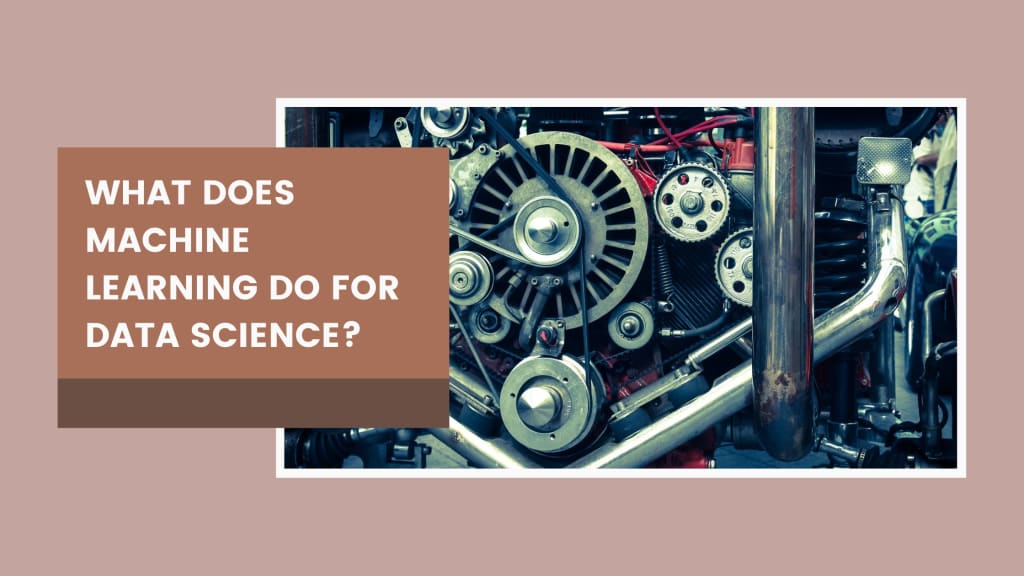
In this article, we are going to see what machine Learning Do For Data Science in an effective manner. Let's get into the Topic.
Introduction
- Among fans of data science, the phrase "machine learning" has recently gained much popularity. It's intriguing to notice that Machine Learning has existed without your knowledge for a long time. Have you ever wondered how YouTube determines which video to show you next? It looks at the videos you're watching, the channel they're from, how long they are, and the topics they cover. Therefore, before suggesting the following video to you on YouTube, all these factors are taken into account. In other words, YouTube "learns" from your viewing habits and suggests movies similar to the ones you are currently watching. For years, you have been seeing examples of how machine learning works.
- You are probably already aware of the wide spectrum of fields that data science encompasses, including machine learning. Data scientists use a range of disciplines and methodologies, including statistics and artificial intelligence, to analyze data and draw pertinent insights. Learn how data science uses machine learning to examine data and glean useful information from it in this article.
- Choose an online Data Science Certification to gain practical experience solving challenging Data Science problems and prepare for a career in the field.
Machine Learning (ML): What Is It?
Simply explained, machine learning is a form of artificial intelligence (AI) or a subset of AI that allows any software application to be more exact and precise in identifying and forecasting results. Machine learning algorithms use historical data to forecast results or output values in the future. Spam filtering, fraud detection, malware threat identification, recommendation systems, and healthcare are just a few examples of the many uses for machine learning.
Why Is Machine Learning So Important?
- Machine learning is once again gaining popularity for the same reasons that data mining and Bayesian analysis have. Things like a rise in the quantity and variety of data, improvements in processing power and cost, and low-cost data storage.
- These elements all work together to produce models that can be easily and automatically built to analyze larger, more complicated data sets and deliver quicker and more accurate results even at sizes. A business also has a better chance of seeing attractive possibilities and averting unknown dangers by creating accurate models.
- Describe data science
- Back in the day, businesses and other organizations could keep the majority of their data in Microsoft Excel Sheets. These data can be analyzed and processed using the most fundamental business intelligence tools. The lack of a lot of data made data handling and management simpler. But as time went on, the volume of data produced each day kept rising.
- This scale of data will be available for evaluation in the future. For processing data of this size, standard spreadsheets and conventional business intelligence tools will be useless. You require a sophisticated data architecture, cutting-edge tools, and technologies to process data of this volume. Data science is used in this situation.
- The goal of data science is to use data as effectively as possible for your company. The impact might manifest itself in a variety of ways, such as YouTube video recommendations or audience tracking data that Netflix uses to develop original programming. Now, in order to complete these duties, you must develop intricate models, write code, and work with data visualization tools.
- According to the Journal of Data Science, data science is "essentially everything to do with data: Collection, Analysis, and Modeling." However, its many applications are its most crucial feature. Data science employs artificial intelligence, deep learning, and machine learning to analyze data and extract useful information from it. Machine learning has many varied applications.
Machine Learning's Importance in Data Science
- Data science is all about drawing conclusions from unprocessed data, which can be done by carefully examining the numerous patterns and trends in the data. In this case, machine learning is useful. However, you must first properly understand the business requirements in order to use machine learning.
- In data science, machine learning algorithms are used when exact predictions about a set of data are required, for as when evaluating whether a patient has cancer based on the results of their bloodwork. This can be done by giving the algorithm many examples, such as people who had cancer or did not, along with the results of each patient's tests.
The Five Basic steps of Machine Learning in Data Science
Step 1: Gathering data
Data gathering is the first step in the machine learning process. In order to solve business challenges, machine learning collects and analyses structured, unstructured, and semi-structured data from any database across systems. It can be written by hand, in a CSV file, PDF, on paper, or as a picture.
Step 2: Cleaning and prepare the data
- Machine learning techniques are used in data preparation to evaluate the data and produce features pertinent to the business issue. ML systems comprehend attributes and the connections between them when they are adequately stated.
- Remember that this is the cornerstone of all data science and machine learning projects. After data preparation, we must clean the data because real-world data is tainted with errors, noise, incomplete information, and missing values.
- We can quickly and automatically detect missing data, do data imputation, code category columns, and remove outliers, duplicate rows, and nulls using machine learning.
Step 3: teach the models
- Model development depends on a variety of variables, including the machine learning algorithm chosen and the caliber of the training data. ML algorithms are chosen in accordance with end-user needs. Model technique complexity, performance, interpretability, computational resource requirements, and speed should all be taken into account for improved model accuracy.
- The training dataset is split into two sections for training and testing once an appropriate machine-learning method has been chosen. It is done to figure out the ML model's bias and variance. A working model that can be further verified, tested, and deployed will be the end result of the model training process.
Step 4: Model prediction
- When addressing model prediction, it is crucial to comprehend prediction errors (bias and variance). With a good grasp of these problems, building correct models and preventing model overfitting and underfitting errors would be simpler.
- By achieving the ideal balance between bias and variance for a successful data science project, you may further reduce prediction mistakes. In recent years, machine learning (ML) and artificial intelligence (AI) have supplanted previous data science disciplines.
- Massive volumes of data are evaluated and analyzed automatically via machine learning. Without involving humans, it automates data analysis and produces current predictions. The data model can be enhanced more and taught to make forecasts in real time. This stage of the data science life cycle involves the use of machine learning techniques.
Conclusion
- Businesses are now utilizing data's potential to enhance their products and services. This article's major objective is to demonstrate how Data Science and Machine Learning complement one another, with Machine Learning facilitating Data Scientists' work.
- In some practical applications, such as speech recognition, online recommendation systems, and fraud detection in all online transactions, data science and machine learning collaborate to produce insightful data. The conclusion that machine learning can analyze data and extract insights will therefore not be false.
Comments
There are no comments for this story
Be the first to respond and start the conversation.