Top 5 Ways Data Science and analytics are Revolutionizing the Insurance Industry
Insurance companies have recently realized the impact of data science and analytics on their bottom line.
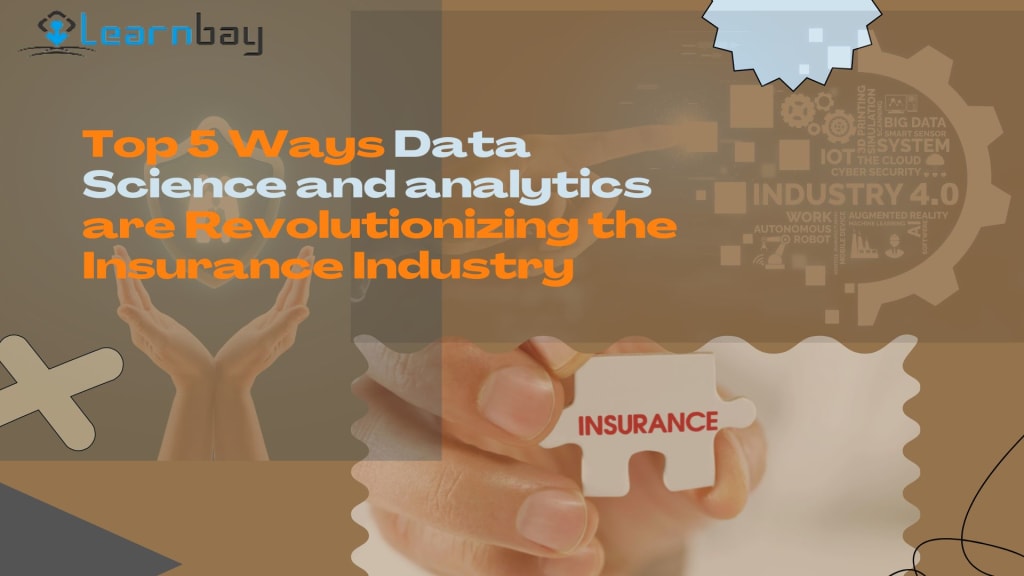
They are now investing in technology that will help them reduce costs, increase revenue and improve customer satisfaction.
In the insurance industry, data science and analytics play an important role in providing better customer service. These two terms provide information about a person or group of people to make decisions on what they need. Data science helps in understanding customer needs by collecting data from different sources and analyzing it using computer algorithms. Analytics helps determine what customers want most by analyzing their needs and behaviors.
Data science and analytics in Insurance
The use of data science and analytics in Insurance can be divided into three categories: predictive modeling, descriptive, and prescriptive modeling.
Insurers use predictive modeling to identify potential risks on actuarial grounds that may lead to loss or damage for their clients through statistical techniques such as regression analysis or neural networks.
Descriptive modeling involves using historical data to describe past events (such as claims rates) or trends (such as claims patterns).
Prescriptive modeling involves using predictive models to make recommendations about managing risks based on what happened in the past. For detailed understanding of these concepts, refer to the data science course in Chennai, accredited by IBM.
5 Ways Data Science Is Changing The Insurance Sector
Insurance is a complicated business, and it would be effective to use data science to improve the efficiency and profitability of the insurance industry. The following are some of the applications of data science applied in the insurance industry.
Automated Insurance Fraud Detection
Detecting fraud is not only necessary for avoiding financial losses but also a valuable contribution to society in general. Nonetheless, some insurers continue to depend only on the claims adjuster's gut instinct for fraud detection, resulting in unreasonably high expenses. With the emergence of big data and increasing access to third-party data to supplement investigations, it is time to shift away from the claims adjuster's gut feeling and toward a more data-driven approach, not to mention the enormous time savings and process optimization advantages.
Fraud detection tools identify fraudulent activities, suspicious relationships, and subtle behavioral patterns using various techniques such as text mining and image screening.
A constant stream of data must be provided to the algorithms in order for them to detect fraud automatically. This information is either from internal records of prior occurrences of fraudulent conduct or from external fraud pools that have been analyzed using sampling methods.
Predictive modeling methods are also used in this case for fraud analysis and filtering. Identifying linkages between suspicious actions aid in the detection of previously undiscovered fraud schemes. In the end, insurers who use these platforms will significantly reduce the number of false claims, resulting in huge savings.
Healthcare Insurance
The practice of health insurance is commonplace worldwide. The costs associated with a disease, an accident, a disability, or death are typically covered. Governments in many nations have been vocal supporters of healthcare insurance policies.
The influence of data analytics applications is unavoidable in the era of rapid digital information flows in this area. The market for healthcare analytics is expanding rapidly on a global scale. The insurance industry is under constant pressure to deliver better services while lowering costs.
For the healthcare insurance industry, various data are gathered, organized, processed, and transformed into insightful knowledge. These data include insurance claims data, membership and provider data, benefits and medical records, consumer and case data, internet data, etc. As a result, factors like cost savings, healthcare quality, fraud detection and prevention, and consumer engagement may all significantly improve.
Customer Lifetime Value (CLV)
Customers' lifetime value (CLV) is a complicated concept that expresses how valuable a customer is to a business as the difference between revenues earned and expenses incurred throughout the entire future relationship with the customer.
Customer behavior data is typically used to estimate the CLV and forecast the customer's profitability for the insurer. The behavior-based models are thus frequently used to predict cross-selling and retention.
Recency, the financial value of a customer to a business, and frequency are considered crucial variables when estimating future earnings. In order to create the prediction, the algorithms gather and process all the data.
This makes it possible to predict whether customers will maintain their policies or cancel them based on their behavior and attitudes. The CLV prediction may also help develop marketing strategies because it puts customer insights at your disposal.
Automated Risk Assessment
An automated risk assessment tool enables you to get to know your customer (KYC), do thorough customer due diligence, and evaluate the risk a customer needs to cover. Adopting risk assessment techniques in the insurance sector ensures risk prediction and reduces it to the bare minimum to reduce losses.
Proper risk analysis takes time for insurers as you have to consider what to cover and who you are insuring.
An automated risk analysis tool allows you to gain real-time access to internal and external data. The risk assessment tool assists you in making quick decisions based on your appetite for risk and data. This accelerates your digitalization and improves the customer experience because the consumer can determine whether or not the insurance policy has already been authorized in seconds.
Claims Automation
Claims automation systems offer one of the biggest options for insurers to position themselves from the market and engage their customers for a lifetime.
Claims automation technologies can assist insurers in optimizing claim workflows from the initial report of loss through settlement, saving time and building stronger relationships with policyholders.
P&C insurers place a high priority on determining damage estimates for a specific claim. The evaluation is frequently based on information gleaned from various sources, including emails and other paper-based or PDF formats, such as damage predictions or police reports. In such instances, an AI algorithm may be trained to extract the relevant data from each document reliably.
Once the claim information is structured, it can be analyzed using robotic process automation (RPA) and artificial intelligence (AI). It decides whether or not the insured has enough coverage. If a claim is evaluated and determined to be low risk, it may be allowed for instant payout or triaged to an adjuster if it is more complex. If a high-risk claim is found, it is forwarded to a special investigative unit for additional review.
Data Analytics in Insurance – The Future Implication
The insurance industry is adopting data-driven models and machine learning techniques to better manage risk and to create products by analyzing massive amounts of data. Overall, the insurance industry is one of the best industries for using predictive analytics and data science as there is plenty of information available about insurance history. This will help improve their profits, reduce wastage in each operation, and increase their client's success rate by getting predictions about the right customers using analytical tools like logistic regressions and decision trees.
If you're from a finance background and looking to move your career in data science, you can sign up for the best data science courses in chennai and become a certified data scientist or financial analyst.
About the Creator
Data science blogger
I am mallikarjun , a data science enthusiast and passionate blogger who loves to write about data science and latest technologies. I always believe in smart learning processes that help people understand concepts better,
Enjoyed the story? Support the Creator.
Subscribe for free to receive all their stories in your feed. You could also pledge your support or give them a one-off tip, letting them know you appreciate their work.
Comments
There are no comments for this story
Be the first to respond and start the conversation.