A Secure Future: Machine Learning's Impact on the Integrity of Tax Systems
Machine learning, a subfield of artificial intelligence, has emerged as a game-changer in the fight against tax evasion.
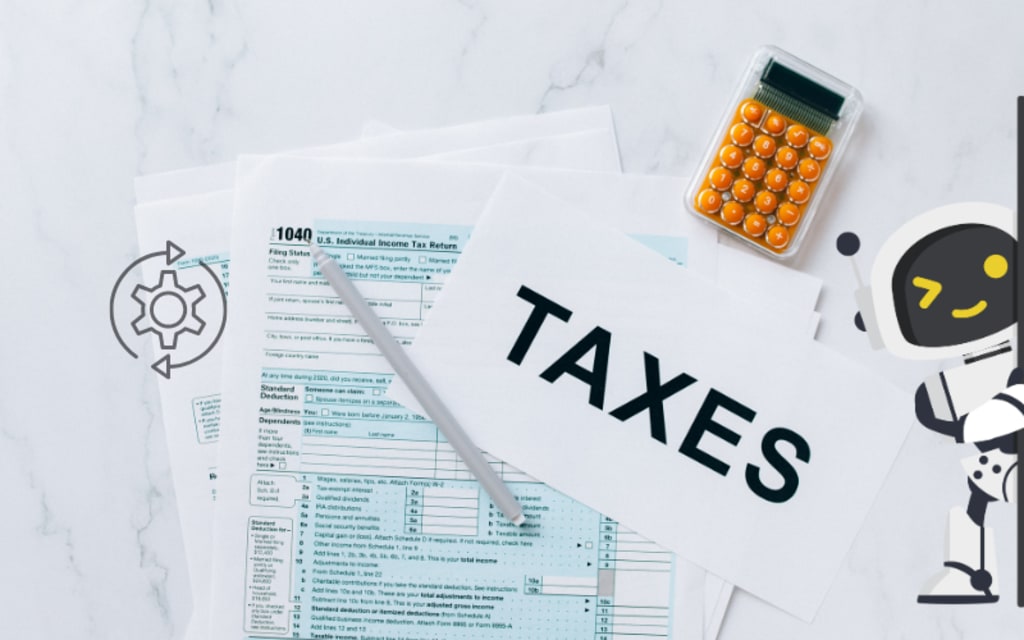
In our rapidly evolving digital landscape, where financial transactions occur at the speed of light and business operations extend across borders, the integrity of tax systems has become a critical concern. Governments depend on accurate and timely tax collection to finance essential services and projects, yet the complexity and sophistication of tax evasion techniques have grown in tandem with technological advancements. This is where the transformative potential of machine learning comes into play – as a powerful tool to fortify tax systems and ensure their integrity in the face of evolving challenges.
The Challenge of Tax Evasion
Tax evasion has long been a thorn in the side of governments around the world, resulting in billions of dollars in lost revenue annually. The battle against tax fraud is exacerbated by the evolving tactics of individuals and organizations seeking to elude their fiscal responsibilities. Traditional methods of detecting tax evasion, such as manual audits and rule-based systems, often struggle to keep pace with the rapid evolution of evasion techniques. These methods are not only labor-intensive and time-consuming, but they also run the risk of overlooking instances of evasion that are cleverly disguised.
Machine Learning's Role
Machine learning, a subfield of artificial intelligence, has emerged as a game-changer in the fight against tax evasion. Leveraging its capacity to process vast datasets, identify patterns, and learn from new information, machine learning algorithms offer numerous advantages for tax authorities:
1. Enhanced Data Analysis
Machine learning algorithms excel at analyzing massive datasets comprising diverse types of information, from financial records to social media activity. By uncovering hidden correlations and anomalies, these algorithms can help tax authorities identify potential instances of evasion that would otherwise remain concealed.
2. Pattern Recognition
Tax evasion often involves intricate patterns and connections that are difficult for humans to discern. Machine learning algorithms excel at recognizing such patterns, even within complex networks of transactions. This capability facilitates the identification of suspicious activities and potential tax fraud.
3. Real-time Monitoring
Conventional tax monitoring approaches often rely on periodic audits, leaving gaps in oversight. Machine learning enables the real-time monitoring of financial activities, allowing authorities to swiftly respond to unusual or suspicious transactions as they occur.
4. Adaptive Learning
Machine learning models continually enhance their accuracy by learning from new data over time. This adaptability is invaluable in combating the ever-evolving strategies employed by tax evaders, as the algorithms can rapidly adapt to new evasion tactics.
5. Risk Assessment
Machine learning can assign risk scores to individuals or businesses based on their financial behaviors and history. This proactive approach enables tax authorities to allocate resources more efficiently by focusing on high-risk entities.
6. Anomaly Detection
Machine learning algorithms can be trained to detect anomalies in financial transactions that deviate from normal patterns. These anomalies could signify potential tax evasion attempts, leading to quicker identification and action.
7. Transaction Monitoring
Machine learning can monitor transactions in real time, flagging transactions that exhibit suspicious characteristics, such as unusually large sums or frequent international transfers.
8. Fraud Prediction
By analyzing historical data, machine learning can predict the likelihood of a tax return containing errors or fraud. This prediction allows authorities to prioritize audits effectively.
Successful Implementations
Several countries have already harnessed the potential of machine learning to bolster their tax systems:
- United States
The Internal Revenue Service (IRS) has adopted machine learning algorithms to identify inconsistencies in tax returns. These algorithms scrutinize historical tax data to forecast which returns are more likely to contain errors or fraud, allowing the IRS to effectively prioritize audits.
- United Kingdom
HM Revenue & Customs (HMRC) utilizes machine learning to identify suspicious patterns in Value Added Tax (VAT) transactions. This technology has substantially reduced the VAT gap by detecting instances of underreporting and evasion.
- Australia
The Australian Taxation Office (ATO) harnesses machine learning algorithms to sift through extensive data from various sources. This enables them to uncover tax avoidance schemes, identify concealed income, and enforce tax compliance with greater efficiency.
The Path Forward
While the integration of machine learning into tax systems offers promising solutions, there are important considerations to address in order to maximize its benefits and ensure a secure future for tax integrity.
1. Data Privacy and Ethical Concerns
One of the foremost concerns in leveraging machine learning for tax enforcement is the delicate balance between data privacy and effective enforcement. Utilizing vast datasets to detect evasion raises questions about individual privacy and the potential for misuse of personal information. Governments and tax authorities must establish robust data protection measures, implement strict access controls, and ensure compliance with relevant data privacy regulations. Transparency in data usage and stringent ethical standards are vital to building public trust in these technologies.
2. Algorithm Transparency and Bias Mitigation
Machine learning algorithms can inadvertently inheritances present in their training data. These biases may lead to unfair targeting, discrimination, or inaccurate assessments. Regular audits and comprehensive reviews of algorithms are essential to identify and mitigate algorithmic biases. Tax authorities should ensure that their machine learning models are transparent and explainable, allowing them to understand how decisions are made and take corrective measures if needed.
3. Collaboration and Interdisciplinary Approach
The successful integration of machine learning into tax systems requires collaboration among various stakeholders, including tax authorities, data scientists, legal experts, and ethicists. An interdisciplinary approach can help ensure that technological solutions align with legal frameworks, ethical principles, and societal values. Regular communication and collaboration between these stakeholders are necessary to navigate complex challenges and make informed decisions.
4. Continuous Learning and Adaptation
Machine learning's effectiveness hinges on its ability to learn and adapt. Tax authorities should establish mechanisms for continuous learning, including the regular updating of algorithms and models to account for new evasion tactics. This dynamic approach enables tax systems to stay ahead of evolving strategies employed by tax evaders, ensuring long-term effectiveness.
5. Hybrid Approach
While machine learning offers powerful tools, it should complement human expertise rather than replace it entirely. Human oversight is crucial in interpreting the outcomes of machine learning algorithms, addressing complex cases, and making decisions that consider broader social and economic implications. A hybrid approach that combines the strengths of machine learning and human judgment is likely to yield the best results.
6. International Collaboration
Tax evasion often transcends national boundaries, requiring international collaboration to combat effectively. Governments and tax authorities can share best practices, data, and insights to collectively address cross-border tax evasion challenges. International partnerships can facilitate the development of standardized approaches to machine learning implementation in tax enforcement, promoting fairness and consistency on a global scale.
7. Public Engagement and Education
Public understanding and acceptance of machine learning's role in tax enforcement are critical for its successful implementation. Governments should engage in transparent communication campaigns to educate citizens about the benefits, limitations, and safeguards associated with using machine learning in tax systems. This engagement fosters a sense of ownership and responsibility among citizens, encouraging them to support the use of these technologies for a secure financial future.
Conclusion
Machine learning holds immense potential to transform the landscape of tax enforcement, enhancing the integrity of tax systems and safeguarding government revenues. By addressing challenges related to data privacy, algorithmic bias, collaboration, transparency, and public engagement, governments can navigate the path forward and harness the full benefits of machine learning in tax enforcement. As technology continues to evolve, embracing these considerations will be instrumental in shaping a secure and prosperous future for tax systems around the world. By combining the power of technology with ethical principles and human expertise, societies can pave the way for an era of resilient and transparent tax enforcement.
About the Creator
NSKT Global
Click here, and learn how you could benefit by letting NSKT Global handle your taxes and accounts!
Comments
There are no comments for this story
Be the first to respond and start the conversation.