Researchers Alert: By 2026, We May Run Out Of Data To Train AI. Then, what?
Do we need to worry?
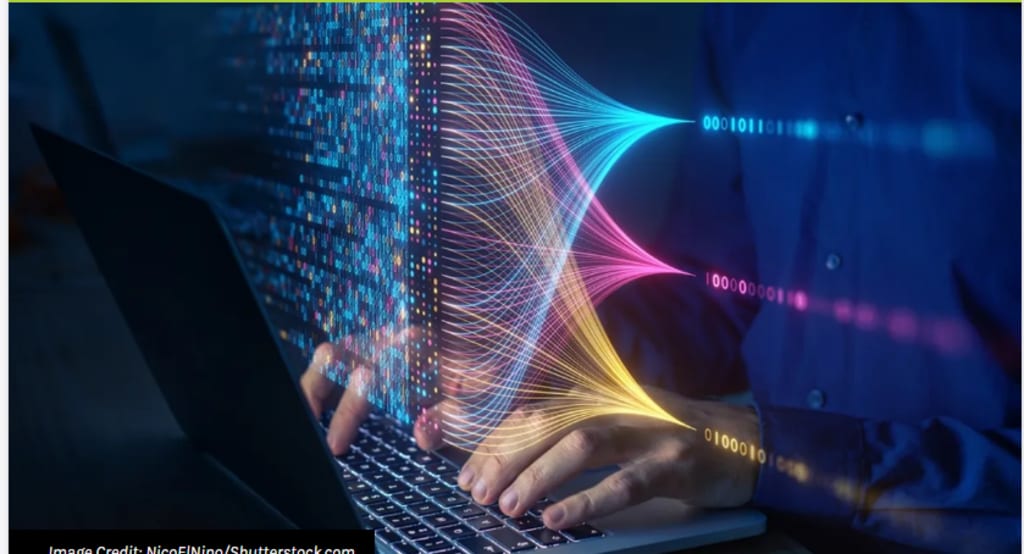
Researchers have cautioned that once artificial intelligence (AI) reaches the pinnacle of its appeal, the industry may run out of training data, which is the fuel that powers extremely potent AI systems. This could potentially change the course of the AI revolution by slowing down the development of AI models, particularly large language models.
But given how many there are online, why is a possible lack of data a problem? Is it possible to mitigate the danger in any way?
The significance of high-quality data for AI
Large amounts of data are required to build strong, precise, and superior AI algorithms. For example, 570 terabytes, or almost 300 billion words, of text data were used to train ChatGPT.
Similar to this, the LIAON-5B dataset, which consists of 5.8 billion image-text pairs, was used to train the stable diffusion algorithm, the brains behind numerous AI image-generating apps including Lensa, Midjourney, and DALL-E. An algorithm will generate results that are erroneous or of poor quality if it is trained on insufficient data.
Another crucial factor is the training data's quality. While it is simple to obtain low-quality data, such as fuzzy photos or social media postings, it is insufficient to train effective AI models.
Text gleaned from social media platforms may contain illegal content or be biased or prejudiced. The model may be able to mimic these things. For instance, Microsoft's AI bot learned to generate misogynistic and racist results when it was trained on Twitter posts.
For this reason, high-quality content is what AI developers look for, including text from books, articles online, scientific papers, Wikipedia, and specific web content that has been filtered. To make the Google Assistant more conversational, 11,000 romance books from the self-publishing website Smashwords were used for training.
Do our data sets suffice?
Because the AI sector has been using ever-bigger datasets to train AI systems, we now have highly effective models like ChatGPT and DALL-E 3. Simultaneously, studies reveal that online data stocks are expanding far more slowly than datasets used for AI training.
If the current trends in AI training continue, a group of researchers predicted in a paper published last year that we will run out of high-quality text data before 2026. Additionally, they predicted that low-quality picture data would run out between 2030 and 2060 and low-quality language data would run out between 2030 and 2050.
By 2030, AI might boost global GDP by up to US$15.7 trillion (A$24.1 trillion), projects accounting and consulting firm PwC. However, the depletion of useful data could impede its advancement.
Do we need to worry?
Although some fans of AI might be alarmed by the aforementioned points, things might not be as dire as they appear. Future developments of AI models remain largely unknown, and there are only a few solutions to mitigate the risk of data scarcity.
One chance for AI developers is to enhance algorithms to make better use of the data they already have.
They will probably be able to train highly effective AI systems with less data and maybe less processing power in the upcoming years. Additionally, this would lessen AI's carbon footprint.
Creating synthetic data with AI to train systems is an additional choice. Stated differently, developers can easily produce the necessary data, tailored to their specific AI model.
Synthetic content is already being used in several projects; it is frequently obtained from services that generate data, like Mostly AI. This is going to happen more often in the future.
Developers are also looking for content from offline repositories and large publishers, as well as content that isn't available for free online. Before the internet, consider the millions of texts that were published. When made digitally accessible, They might offer a fresh source of information for AI initiatives.
News Corp, a global leader in news content ownership with a large portion of its content protected by paywalls, recently announced that it was in talks to work with AI developers on content agreements. These agreements would compel AI companies to pay for training data, which they have thus far primarily obtained for free by scraping it from the internet.
Some content creators have sued Microsoft, OpenAI, and Stability AI to stop their work from being used without permission to train AI models. Paying people for their work could contribute to reversing some of the power disparities between AI companies and creatives.
What is it if it's not outright theft?
Media-to-text Although AI is intrinsically very complex, even those of us who are not computer scientists can understand it conceptually.
It's important to take a step back and consider how individual artist styles can enter and exit the black boxes that power systems such as Lensa in order to fully appreciate the benefits and drawbacks of Lensa.
In essence, Lensa is a simplified and personalized front-end for the open-source Stable Diffusion deep learning model. It gets its name from the fact that it generates creative output through a mechanism known as latent diffusion.
Here, the word "latent" is crucial. A latent variable in data science is an attribute that can be inferred from things that can be measured but cannot be measured directly.
During the development of Stable Diffusion, a vast quantity of image-text pairs were provided to machine-learning algorithms, which trained themselves to find billions of possible connections between the images and captions.
This produced a sophisticated body of knowledge, none of which can be understood by humans directly. While its outputs may seem to us to be "modernism" or "thick ink," Stable Diffusion perceives a universe of connections and numbers. the numbers derived from the original image-text pairs are the source of all this intricate mathematics.
The system absorbed both image and description data, allowing us to navigate the vast array of potential outputs by inputting insightful prompts.
Comments (1)
Overall, it offers valuable insights into the evolving landscape of AI and its reliance on data. Just Say Excellent