AI startups transforming dissemination of biomedical science
It takes two to tango
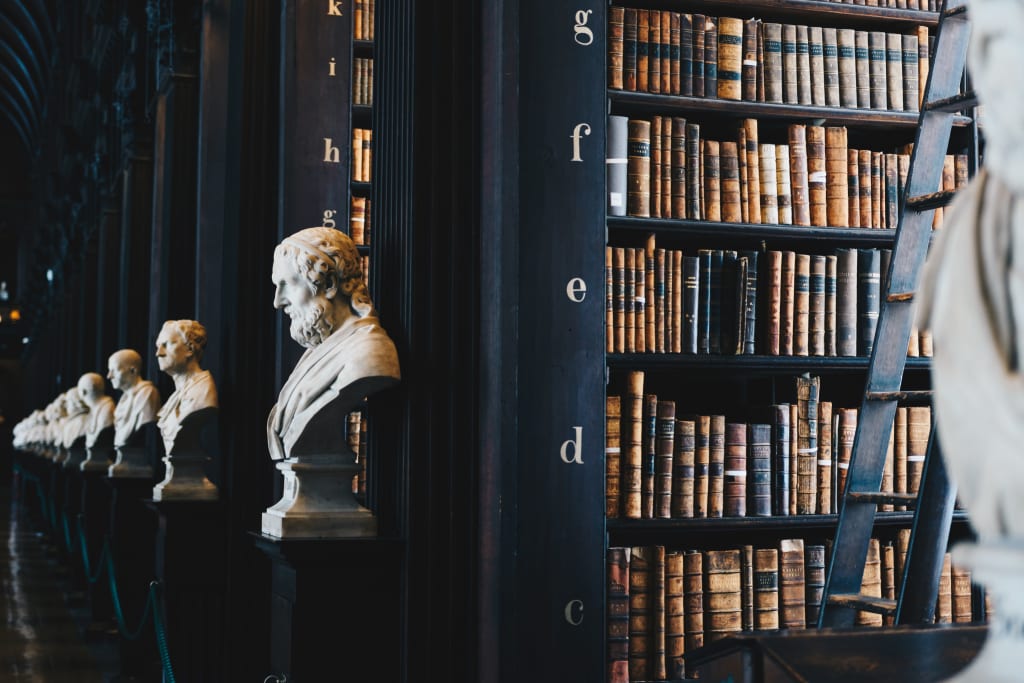
Where does research begin?
When it comes to producing biomedical scientific knowledge (research, research papers, and lead-generators for pharma) the best thing that describes the relationship between academia and pharma is a tango. A dance that requires two partners moving in relation to each other, sometimes in tandem and sometimes in opposition.
Like real tango - the result of a combination of Waltz, Polka, Mazurka, Schottische, Habanera, Candombe, and Milonga - like so the academia-pharma tango is the result of a combination of university researchers, professors, nerds, medical doctors with PhDs, corporate scientists and corporate white-collar executives.
In this academia-pharma tango, everything starts as an early stage research process (drug-biomarker discovery) in a university lab or in university spinoff entity or in a small biotechnology company and is sponsored by the government or by pharma or by both.
After that, and through a complicated and elaborated process tons of data are produced and kept hidden behind a firewall (negative results or/and hidden results) while novel preliminary results are seen by few during only conferences (abstracts, posters and power point presentations).
At the end of this process, and on average after 2–5 years, flattering and only positive results of the early stage research will be published (papers) and presented to the public after going through the peer review process. These papers, once published, are usually considered pharma lead-generators for choosing future drug candidates for further drug-biomarker development.
The peer review process ✍🏻
The academic peer review took its first steps in 1665 in order to successfully carry out its mission to ensure that “the honor of X author’s invention will be inviolably preserved to all posterity”, so it was determined that “the Y article in the Society’s Science Transactions should be first reviewed by some of the members of the same (reviewers)”.
This system - a process at the heart of all science - has remained essentially unchanged since 1665 (!!!!???) and nowadays it is the method by which:
- papers are published for dissemination of scientific knowledge,
- grants are allocated,
- academics are promoted, and
- Nobel prizes won.
But as Richard Smith - a British medical doctor, editor, businessman, and chief executive of the BMJ Publishing Group for 13 years among other things - wrote in his article:
“peer review it is compared with democracy, a system full of problems but the least worst we have”.
In fact, in 2005 the legendary Greek-American physician-scientist-writer Stanford epidemiologist John Ioannidis wrote a paper - which has become the most widely cited paper ever published in the journal PLoS Medicine - and examined how issues currently ingrained in the scientific publishing process indicate that at present:
“most published findings are likely to be incorrect”.
After Ioannidis, more than a decade later, the UK–based medical writer Richard Horton and editor-in-chief of The Lancet put it only slightly more mildly:
“much of the scientific literature, perhaps half, may simply be untrue”.
As a matter of fact, according to Richard Smith and Christopher Tancock (Editor-in-Chief of Elsevier) the peer review process is:
- slow and expensive,
- inconsistent,
- with reviewers sometimes turned out to be fake, overworked, underprepared, not consistent and rarely paid for,
- with agencies that “handle the peer review process” for authors,
- with citation manipulations,
- with ghostwriters,
- with flagrant conflicts of interest,
- with publication bias: a process where negative results go unpublished, together with small sample sizes, with tiny effects and invalid exploratory analyses, and
- with an obsession for pursuing fashionable trends of dubious importance, that has allowed science to take a turn towards darkness.
In other words, as a result of all above, the replication or reproducibility crisis in the scientific publishing industry has emerged.
Replication or reproducibility crisis
In 2011, a group of researchers at Bayer decided to look at 67 recent drug discovery projects (early-stage research) and they found that in more than 75% of cases the published data did not match up with their in-house attempts to replicate. These were not studies published in fly-by-night oncology journals, but blockbuster research featured in Science, Nature, Cell, and the like (Source: "House of Cards: Is something wrong with the state of science?" by Harvard Edu).
As a matter of fact, the medical literature is considered by its own practitioners to be the least reliable. Interestingly, chemists, physicists and engineers, are among the most confident in the literature of their own field.
Moreover, in a paper published in 2012 ("Raise standards for preclinical cancer research"), one of the authors Glenn Begley - a biotech consultant working at Amgen - said that during his decade of cancer research he tried to reproduce the results of 53 so-called landmark cancer studies (landmark papers are highly influential papers that have substantially changed the practice of medicine).
But, after his team wasn’t able to replicate 47 out of these 53 studies - even after repeating 50 times the experiments in each study - he realised that in the originals studies the authors had repeated the experiments only 6 times, finding positive results only once and publishing only this positive result.
In fact, under our current scientific publishing system, most of the information about failures “the boring” negative results, are just brushed under the carpet and this has huge ramifications for the cost of replicating research.
Researchers build theories on the back of landmark cancer studies, since they take them for valid and investigate the same idea using other methods. So, when they are led down the wrong “research path”, then huge amounts of research money and effort are being wasted, and the discovery of new medical treatments is being seriously retarded. And unfortunately, every month there is some kind of news about problems with replication issues in the scientific publishing industry (Source: "1,500 scientists lift the lid on reproducibility").
Moreover, a huge amount of early-stage research gets presented only at conferences (abstracts, posters and presentations) - and it is estimated that only half of it appears in the academic literature - since these studies presented only at conferences are almost impossible to find or cite since very little information is available online.
Additionally, a systemic review done in 2010 looked for papers investigating at what happens to all conference material. In particular, in the 30 separate studies they found, they then subsequently looked whether negative results on conference presentations disappear before becoming fully-fledged academic papers. Interestingly, it came out that in the vast majority unflattering negative results are more likely to go missing (Source).
Furthermore, specific academic literature can be ghost managed, behind the scenes, to an undeclared agenda. In reality, some academic articles are often written by a commercial writer (ghostwriter) employed by pharma, with an academic’s name placed at the top to give imprimatur of independence and scientific rigor. Often, these academics have had little or no involvement in collecting the data or drafting the paper.
And here is where the problem only gets bigger.
Developing a new prescription medicine that gains marketing approval is estimated to cost drug makers something like $2.6 billion, with overall success rates 5.1% for cancer drugs and 11,9% for all other drugs (from phase 1 to FDA approval).
Furthermore, the entire process of drug development (journey from lab to shelf) takes 10 to 15 years, and for each $1 billion spent on R&D the number of new medicines approved has halved roughly every nine years since 1950. On average, the thirty large and small pharmaceutical and biotech companies got ONLY 11% of their 2017 revenue from drugs developed within the past five years. (Source)
Accordingly, if early-stage research is where novel hypothesis for future drug-biomarker candidates are being formulated - but once early-stage research goes through the “bottleneck of the peer review process” comes out as a replication crisis - where do researchers are supposedly going to get new lead generators for further drug-biomarker development?
And since drug-biomarker development - from early stage to clinical phase - is an ongoing research activity strictly correlated with published findings (papers) and unpublished data (omics, lists, graphs, images, ppt etc) hidden behind a firewall, how are we going to overcome the replication crisis and the decline in pharmaceutical R&D efficiency along with their healthcare, economic and political consequences?
Well, while academia and pharma are involved in this “whose fault is it” debate regarding data reproducibility, I think innovation and digital transformation of the old process of disseminating biomedical scientific knowledge it seems to be the only solution we have at the moment.
Hypothetical scenario of an early stage research
Imagine now this hypothetical scenario, where two researchers one from academia and one from pharma - let's call them Black and White - decide to work together on the same hypothetical drug candidate, named Red.
Early mid-stage research - after target and lead identification and validation - starts by testing Red on cell lines (in vitro studies) and that is the beginning of the preclinical studies. Considering that Black and White are working in a state-of-the-art laboratory, then more likely after six months they will have produced their first “real life” results, meaning some negative and some positive results.
In consequence - after obtaining their first positive and negative results with P-value ideally less than 0.01 - Black and White decide to present them at conferences, congresses, seminars and meetings, and for that reason, they prepare posters, abstracts, videos, data sets (omics data) and powerpoint presentations.
Interestingly, when these results will be presented for the first time in the form of posters, abstracts and powerpoint presentations, that is the closest we have to REAL TIME results (6 months after the beginning of the studies, let's call this t0=beginning).
Unfortunately, and until very recently, these huge amounts of preliminary data couldn’t be found online. Moreover, Black’s and White’s results at time1 = t0+ 6 months are strictly correlated with the technological and scientific advances at that precise time.
Meaning, if Black and White have to wait for example another 3 years in order to complete all their preclinical studies and publish all their results, then more likely by the time their paper is published (time2 = t0+ 3 years) their results would be probably “old enough”, not reflecting the actual technological and scientific advances 3 years after the beginning of their studies.
Now, after Black and White have finally presented their positive and negative results at a conference, their next step is to continue their studies by testing Red on mouse models (in vivo studies). The in vitro and in vivo experiments will determine their preclinical phase of drug-biomarker discovery.
And here comes the best part, if they don’t test Red in vivo and decide to publish only the in vitro studies, more likely their findings are not going to be accepted by a high impact journal (where blockbuster research is featured), and sometimes these results might just remain positive and negatives results on an abstract and get lost in some huge fire and humidity resistant archive.
However, even if they decide to test Red in vivo and wait for a minimum of 2.5 years (if everything goes well) to complete all the preclinical studies, they will still have to prepare themselves for a publishing journey of
- power bias,
- conflicts of interest,
- fashionable trends of dubious importance and finally
- journal shopping, a process where scientists submit first to the most prestigious journals in their field and then working down the hierarchy of impact factors.
This creates an endless cycle of submission, rejection, review, re-review and re-re-review that seems to eat up months of Black’s and White’s lives, that interferes with their job and slows down the dissemination of biomedical scientific knowledge.
Conclusion: the old peer review process may be inhibiting innovation in drug-biomarker development.
AI startups for biomedical science 🕵🏻
Luckily for White and Black, the solution to their problems comes from data-driven startups, employing ML and AI for data aggregation, analysis and dissemination during drug development. Let's see some examples.
Data4Cure in California offers a Biomedical Intelligence Cloud platform using ML and AI applications built on top of the largest repository of semantically linked biomedical data (genomic, phenotypic and clinical datasets) and literature, allowing researchers to identify new targets and biomarkers, repurpose drugs and identify disease pathways. Central to this platform is a dynamic biomedical knowledge graph called CURIE™ spanning over 1 billion biomedical facts and relations continuously inferred from thousands of datasets (both public and customer-specific) and millions of publications. Genialis in Texas uses AI to analyse multi-omics next-generation sequencing data allowing researchers to reveal previously unseen patterns across large, heterogeneous datasets. Their goal is to leverage RNA sequencing and clinical trial outcomes data to model gene signatures that stratify patients based on predicted drug response. Evid Science in California has a patented AI, which they claim can read up to 25 million articles in an hour, and has already processed the publicly available medical literature across all endpoints, interventions and therapy areas and updates nightly, enabling to make faster, smarter, evidence-based decisions. Innoplexus in Frankfurt is a consulting-led technology and product development company focusing on big data and analytics, using AI to generate insights from billions of disparate data points from thousands of data sources including publications, clinical trials, congresses and theses. (Source)
Causaly Inc in London offers a semantic AI-platform which reads collections of scientific articles and extracts causal associations through linguistic and statistical models dealing with THE MOST difficult biomedical challenge: increase productivity in literature reviews by filtering out false positives.
Datavant Inc in San Francisco employs AI to aggregate and analyse biomedical data to lower the time, cost and risk of drug development, and specialises in breaking down silos and analysing health data securely and privately.
Quertle in Nevada enables unparalleled discovery of literature through AI-powered searching, integration, organisation and presentation including predictive visual analytics, covering journal articles, patents, clinical trials, treatment protocols and much more. Linguamatics in Cambridge utilises AI to extract and analyse text (mining software) from large document collections: basic research, patents analytics, drug safety and pharmacovigilance, precision medicine, voice of the customer, real world data, clinical trial analytics, regulatory compliance, clinical research and many more. Owkin in New York develops ML to connect medical researchers with high-quality datasets from leading academic research centers around the world and applies AI to research cohorts and scientific questions. Plex Research in Boston has a unique AI search engine technology that offers a search engine that connects all types of scientific data (broad array of public sources and databases) and allows organisations to make the most use of their internal scientific data by connecting organisation’s own proprietary data and algorithms with the public data. (Source)
Percayai in Missouri uses AI to organise and prioritise data in a contextual manner, enabling interactive 3D diagrams illustrating biological information, allowing researchers to rapidly generate testable hypotheses from complex, omic and multi-omic data sets. Sparrho utilises AI to curate - in combination with human expertise - millions of scientific papers from thousands of publications, allowing researchers to stay up-to-date with new scientific publications and patents. They have 60M+ research articles/patents aggregated, they have indexed 50K+ unique data sources and they have 18K+ content curators (scientists, researchers, PhDs, teachers) across the globe. Molecular Health in Heidelberg offers a cloud-based MOLECULAR HEALTH DATAOME platform to analyse molecular and clinical data of individual patients against the world’s medical, biological, and pharmacological knowledge, to drive more precise diagnostic, therapeutic and drug safety decisions. OneThree Biotech in New York utilises AI to integrate and analyse data from over 30 types of chemical, biological and clinical data allowing researchers to generate new insights across the drug development pipeline. (Source)
Euretos in Zuid-Holland The Netherlands uses natural language processing to interpret research papers (2- 2.5 million new scientific papers are published each year in about 28,100 active scholarly peer-reviewed journals), but this is secondary to the 200-plus biomedical-data repositories it integrates. Finally, OccamzRazor in California uses AI to transform all available data about Parkinson's disease into machine-readable graphs (Human Parkinsome), by consuming and analysing unstructured and structured biomedical datasets (e.g., published literature, preclinical and clinical trial results).
Not bad after all for Black and White!
Thank you for reading 👓💙
And if you liked this post why not share it?
Comments
There are no comments for this story
Be the first to respond and start the conversation.