Why and How Implement a data architecture?
changes in the business or in technology can be the impetus for developing or updating a data architecture.
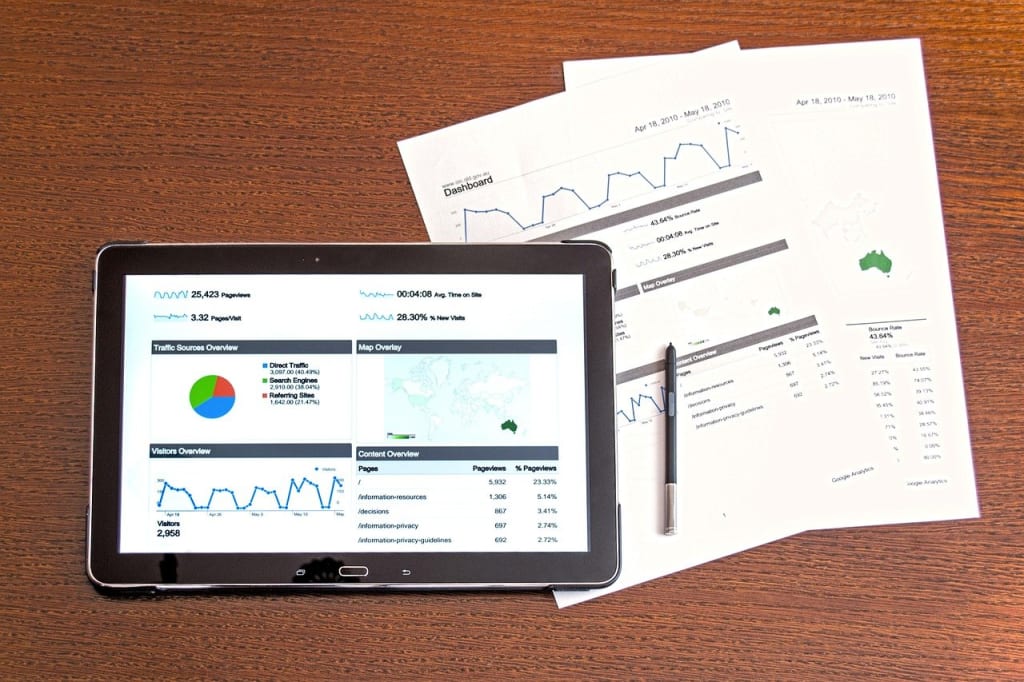
Changes in the business or in technology can be the impetus for developing or updating a data architecture.
Data flows can be reexamined when a key element of an organization's IT or analytics systems is changed. Additionally, the transition to cloud technology provides a mechanism to change data flows without having to "lift and shift" systems. Changes may instead be made on an application- or project-by-project basis.
According to Garrood of PA Consulting, "Painting that image of what the advantages can look like is part of the data architect's duty." But it's also to determine what changes should be made and what new flows should be added to the pipeline.
This is also supported by the shift from data warehouses to data lakes, as data should no longer be tied to particular applications.
According to Roman Golod, CTO and co-founder of data operations company Accelario, "Firms have a lot of new sources and data." They must transition to new technologies, such as web services and the cloud, as well as continuous integration between many sources.
Golod observes that the majority of his clients—perhaps 80%—remain dependent on on-premise solutions. But hybrid technologies or the cloud are increasingly where new capabilities are coming from.
This enables companies to take another look at that crucial blueprint or data flow, find new data sources, and perform more sophisticated analytics, ML, and AI. Organizations must first organize their data, though, before doing so.
Although data quality and master data management may not technically fall under the purview of data architecture, they are still essential to delivering the business outcomes of an architectural project. Connecting disparate systems can frequently uncover data quality issues that were previously undetected, according to professionals who have worked on large-scale data architecture projects. And if the organization wants to have confidence in the conclusions drawn from advanced analytics or ML/AI tools, it is crucial to have a clear knowledge of which records are the master, or "golden," data.
This is especially true in cases where an organization has a significant number of systems, some of which may be outdated designs or systems with accumulated technical debt. One of the clients of KPMG's firm, in the oil and gas industry, has had more than 1,500 data integrations, says Whitfield. For instance, integrating those data points into a data lake poses issues with compliance, practicality, and data standards.
According to the information kind, "that information spectrum has to be managed, and as a result, the underlying datasets also have to be controlled," he claims. "At one end, you have data that must be tightly regulated, highly controlled, extremely consistent, and, in general, unaltered. On the other hand, you give data scientists access to sizable data sources and allow them to do whatever they want with the information. In actuality, it is a difficult effort for the data architecture to support both sides of that range.
Data specialists advise taking an incremental approach or considering data architecture in the context of specific projects or business cases. If not, the task could become overwhelming and fail to produce business advantages. However, this still needs to be connected to the broader data model that the company is aiming for. This will always be difficult because there are too many little projects, each with its own risks, data standards, and information silos.
A business case and data architecture
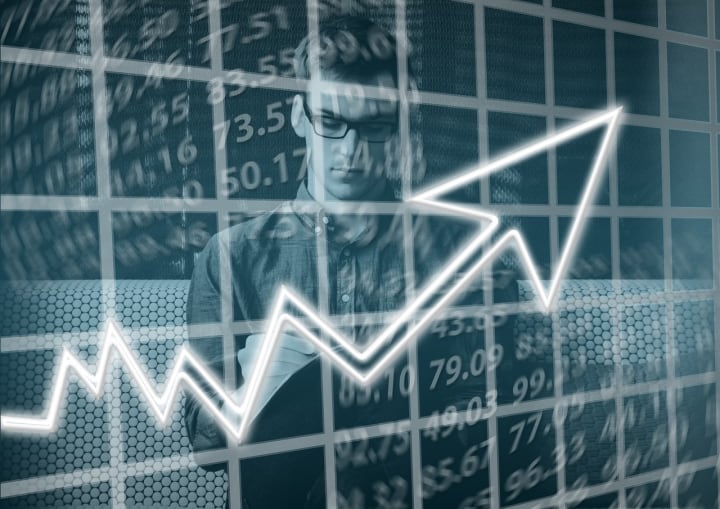
But making an investment in data architecture can pay off significantly, and even quickly.
Businesses will be better positioned to benefit from new and emerging platforms and applications, such as AI and the cloud, as well as make greater use of the data they already have. Additionally, as Dufrain's Bowes notes, a modernized data architecture allows businesses to have a more complete picture of their consumers by, among other things, connecting data from cloud and software-as-a-service (SaaS) systems to pre-existing data warehouses.
Organizations can use data architecture to manage technological debt and make sure that their data retention and acquisition policies are legal. But in the end, it all comes down to maximizing the value of the data that the company has already paid for to gather.
According to PA's Garrood, "it is fundamentally there to mimic the world we perceive, and to portray that world in some way." "At its core, modeling entities and their connections continues to be important. Being clear about your goals is fundamental, and it all boils down to that.
But this also requires a commitment to using the new data and insights, as well as corporate leadership, continuing administration, or even curation.
Without the proper data leadership in place, a data architecture—a beautifully documented thing—is useless, according to Whitfield of KPMG. Better insights undoubtedly have value, and there are numerous business prospects there. However, the physical organization of our data is only a small component of that. It involves the business case leadership, the appropriate operating model, governance structure, tooling, and finally the appropriate culture.
Comments (2)
Well thought out and well written
Very well written