Unraveling Emotions: Harnessing AI for Sentiment Analysis
This blog explains how AI helps in Sentiment Analysis
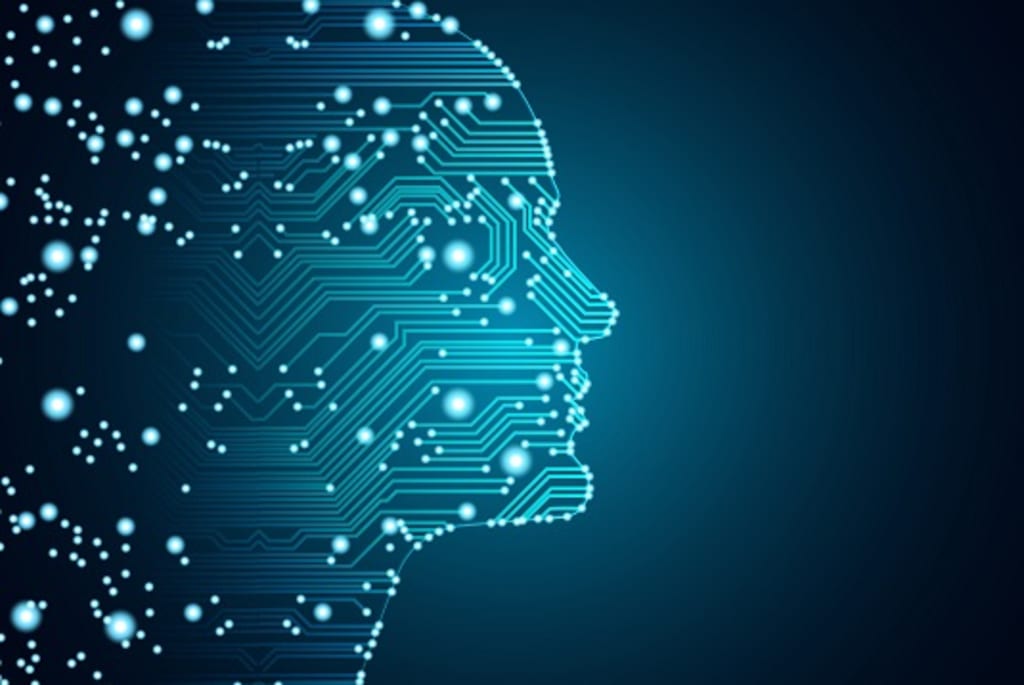
Nowadays, because of the online interconnected digital world, which echoes people's opinions and emotions over the social media, online reviews, and customer forums, the need to get an insight into the feelings and emotions behind the textual data has become very important. Here we go—the sentiment analysis is one of the dominant applications of artificial intelligence (AI), through which organizations can now mine unstructured text data with much success assigning emotions to users and customers with unprecedented precision. This blog takes you on a journey into the intriguing world of sentiment analysis and uncovers the role AI plays in the growing impact of its ability to capture and interpret human emotions in the digital space.
Decoding Sentiments with AI
Sentiment analysis, opinion mining or the automatic process of finding, extraction and classification of emotions contained in texts is one of the widely applied techniques for this textual data. This is done by applying natural language processing (NLP), which is combined with machine learning algorithms to analyze texts and identify if the sentiment conveyed is positive, negative or neutral.
The applications of sentiment analysis are diverse and far-reaching, spanning across various industries and domains:
1. Business Intelligence: Business entities can take advantage of the customer feedback on different online channels such as reviews, comments on the social media platforms and rankings to make conclusions about customers' views the likes, and satisfaction. Sentiment analysis aids businesses in identifying market trends, instead of what they thought was true, and enables them to refocus their resources on manufacturing products and services that meet customer expectations.
2. Market Research: Sentiment analysis gives marketers the upper hand to analyze consumer's mood state Online towards products, brands, and advertising of the products on a real-time basis. Since social media platforms are the place where customers meetings nowadays, researchers can listen to people's comments and conversations that may shape consumer opinion and show industry trends. Thus, with those data, marketers may do research and develop strategies and products.
3. Customer Support: AI-powered sentimental analysis tools can analyze customer tickets including chat or email messages and rank different issues based on which ones should be addressed first and on the expressed emotional intensity. Through finding out times of dissatisfaction or frustration, companies can give a chance to their customers to express dissatisfaction swiftly and escalate them in order to solve the problems. This leads to improved customer satisfaction and, as a result, increased customer retention.
4. Financial Analysis: Sentiment analysis has become an in-demand tool in financial positions, to assess textual accessories such as news, articles, and social media posts for an idea of investor’s sentiments and market patterns. Though the exact formula to gauge market sentiment is variable, and techniques are dynamic, it might provide some insights into investor's psyche, thus aid in decisions making and attenuating the risk from market volatility.
AI-Powered Sentiment Analysis Techniques
AI-powered sentiment analysis relies on a variety of techniques and approaches to analyze and interpret textual data:
1. Rule-Based Systems: Rule-based sentimental analysis systems leaning on linguistic predefined rules and patterns use to separate textual information into three categories: positive, negative, or neutral sentiments. The strength of the simple-to-implement rule-based systems might come at the expense of flexibility and versatility to capture the intricacies of language and context, and these systems might not be able to forcefully challenge domain experts.
2. Machine Learning Algorithms: Machine learning based sentiment analysis algorithms is trained with labeled training data, so it can automatically label text with a certain category of sentiment. The most commonly used supervised learning algorithms for the purpose of sentiment classification are Support Vector Machines (SVM) and Naive Bayes classifiers. The newest approaches involving the use of deep learning, such as Recurrent Neural Networks (RNNs), Convolutional Neural Networks (CNNs) have already become well-established in this domain because they allow for the use of complex linguistic patterns
3. Aspect-Based Sentiment Analysis: Sentiment analysis of basic aspect provides more than just general polarity of users’ sentiment towards a specific characteristic: it is a way of analyzing why each mentioned feature is positively (or negatively) considered. That particular feature gives a more detailed reading thus corporations can go ahead and identify separate domains that they can either optimize or improve.
Challenges and Considerations
While AI-powered sentiment analysis holds immense potential for extracting valuable insights from textual data, several challenges and considerations must be addressed:
1. Contextual Understanding: Recognizing the subtleties of language and situation is a major task for sentiment analysis systems. This task mainly applies in cases of sarcasm or irony and cultural context that may misguide human judgment of what sentiment should be attached to a message being analyzed.
2. Data Quality and Bias: Sentiment analysis models draw information that they can process only from the data sets that were used to train them. Incomplete or biased training data may contain underrepresentation of certain population, language or even cultural stereotypes what can lead to unfair results and inaccurate sentiment prediction.
3. Multilingual Analysis: Sentiment analysis tends to be more challenging in multi-lingual settings, where applying language variations and culture factors also matter. Text sentiment analysis tools powered by artificial intelligence should have an ability to handle and interpret text in multiple languages in order to give precise views in any of the linguistic contexts.
Conclusion: Empowering Data-Driven Decision-Making
In summary, AI-driven sentiment analysis is a transformative tool that is bringing new horizons for organizations by helping them with the actionable insights that are based on textual data, which can make decisions based on data in different spheres. Accurate sentiment analysis allows businesses to become familiar with what customers feel, what trends exist in the market, and what reputation the brand has, thus fueling innovation, progressiveness, and competitiveness.
With the evolution of AI, sentimental analysis becomes the key to the future, opening up frontiers of higher accuracy and interpretability, as well as creating endless ways of tailoring and personalization. By making use of AI in sentiment analysis, the technology is able to mine the latent value of corporate textual data so that the companies can pick up or have a more accurate perception of people’ feelings within the digital space.
want to explore the analysis space explore data science course with placements.
About the Creator
Fizza Jatniwala
Fizza Jatniwala, an MSC-IT postgraduate, serves as a dynamic Digital Marketing Executive at the prestigious Boston Institute of Analytics.
Comments (1)
Great job.