How does AI learn?
Artificial intelligence
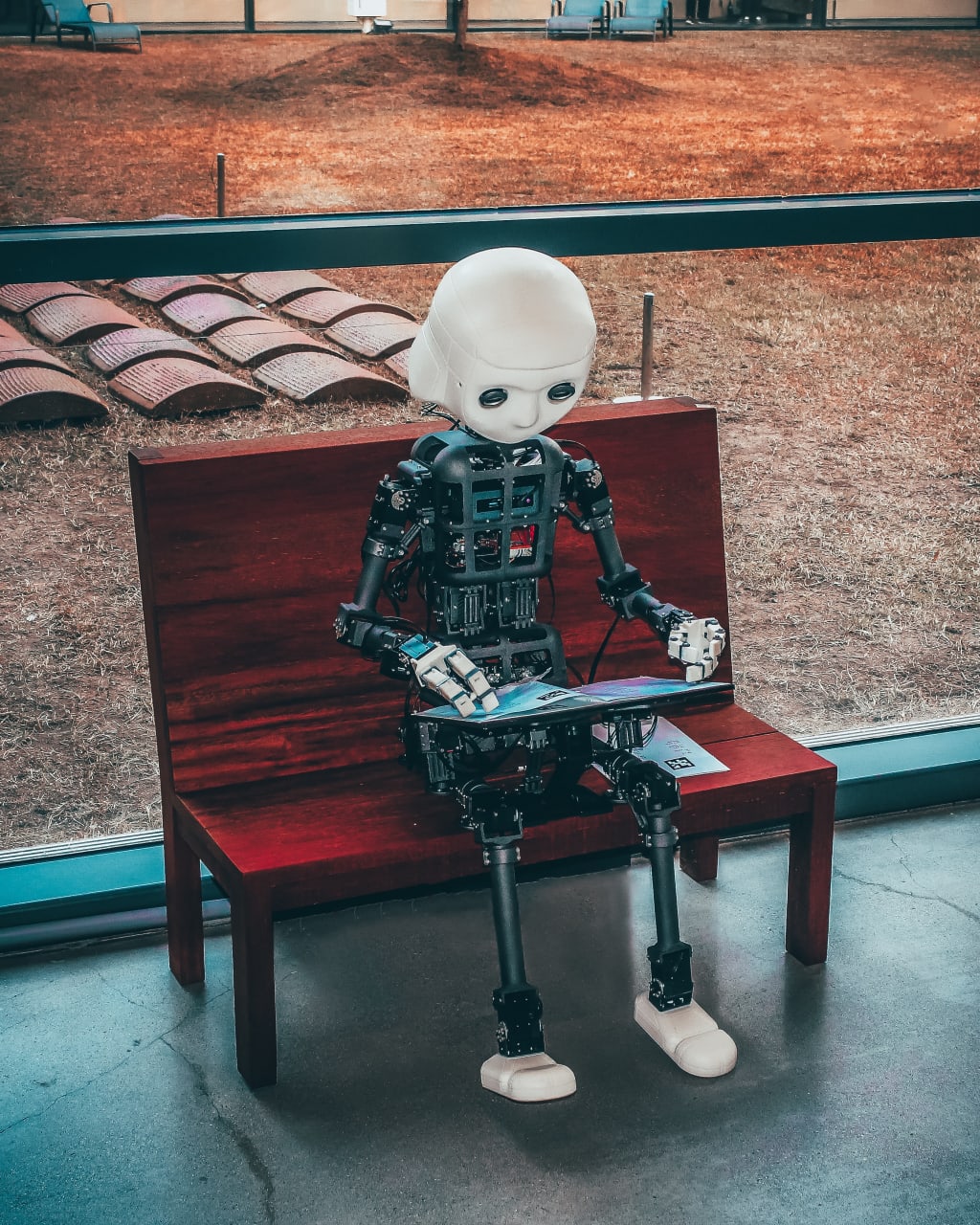
Today, artificial intelligence aids in the diagnosis of patients, the operation of commercial aircraft, and the traffic forecasting of cities. But whatever these AIs are doing, it's likely that their creators—the computer scientists—don't fully understand how they're doing it. This is so because artificial intelligence frequently learns on its own, building its own set of rules and tactics based on a limited set of instructions. There are many different ways to create self-teaching programs, so how exactly does a machine learn? But they all rely on the three fundamental varieties of machine learning: unsupervised learning, supervised learning, and reinforcement learning. Consider a scenario where researchers are attempting to glean information from a set of medical data that includes thousands of patient profiles. Unsupervised learning is the first topic. The analysis of all the profiles using this method would be perfect for identifying commonalities and insightful patterns.
Perhaps a treatment causes a certain set of side effects, or maybe a certain group of patients present with a disease in a similar way. Without the aid of a human, it is possible to use this all-encompassing approach to find emerging patterns and find similarities between patient profiles. Consider, however, that physicians are seeking a more focused answer. These doctors want to develop an algorithm for identifying a specific condition. To start, they gather two sets of information: test results and medical images from both healthy and patients with the condition. Then, they enter this data into a program made to find characteristics shared by the ill patients but not by the healthy patients. The program creates an algorithm for diagnosing future patients by assigning values to each feature's diagnostic significance based on how frequently it observes it. But unlike unsupervised learning, the next step is actively controlled by medical professionals and computer scientists. The final diagnosis will be made by doctors, who will also evaluate how accurately the algorithm predicted the outcome. The program's parameters can then be modified to increase accuracy using the updated datasets, according to computer scientists. Supervised learning is the name given to this practical method. Let's say these medical professionals want to create a new algorithm to suggest treatment courses. The doctors choose reinforcement learning because these plans will be implemented gradually and they might change based on how each patient responds to treatments.
To learn more about the most efficient drugs, doses, and treatments, this program employs an iterative methodology. Then, to create each patient's specific, ideal treatment plan, it compares that data to their individual profile. The plan for each patient can be continuously updated as the course of treatment develops and the program receives more feedback. There is no inherent advantage between these three methods. Even though some require more or less human involvement, each one has unique strengths and weaknesses that make them best suited for particular tasks. However, by combining them, researchers can create sophisticated AI networks where different programs can guide and instruct one another. For instance, when our unsupervised learning program identifies patient groups that are similar, it might transmit that information to a connected supervised learning program. This information could then be incorporated into the program's predictions. Or perhaps dozens of reinforcement learning applications will simulate potential patient outcomes in order to gather feedback on various treatment options. These machine-learning systems can be made in a variety of ways, but the ones that most closely resemble the interactions between brain neurons appear to be the most promising. Millions of connections can be made by these artificial neural networks to handle challenging tasks like speech recognition, language translation, and image recognition.
However, as these models become more self-directed, it becomes more difficult for computer scientists to understand how these self-taught algorithms arrive at their solution. The topic of increasing machine learning transparency is currently being researched. However, as AI becomes more present in our daily lives, these mysterious choices affect our jobs, our health, and our safety more and more. In order to teach machines to investigate, bargain, and communicate, we must also think about how to teach them to operate ethically.
Comments
There are no comments for this story
Be the first to respond and start the conversation.