Building Trust and Transparency in Data Science Practices
Data Science Practice
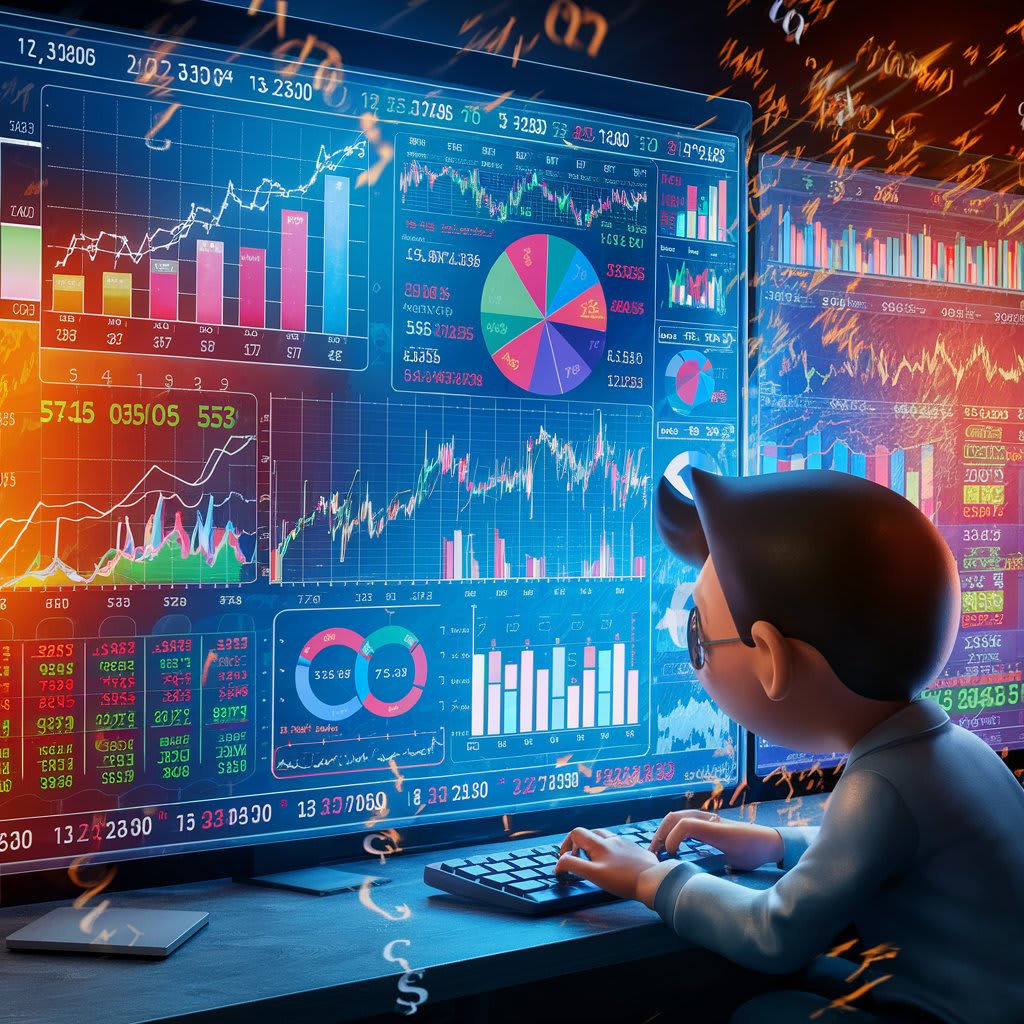
Table of Contents:
1. Introduction
2. The Importance of Data Privacy and Security
3. Implementing Robust Data Governance Frameworks
4. Ensuring Compliance with Data Protection Regulations
5. Embracing Explainable AI for Transparent Decision-Making
6. Fostering Interpretability in Machine Learning Models
7. Communicating Model Limitations and Uncertainties
8. The Role of Data Scientist Training and Placement in Promoting Ethical Practices
9. Cultivating a Culture of Responsible Data Science
10. Future Trends and Considerations
11. Conclusion
Introduction
In today's fast-moving world of data science, distilling useful insights from vast lakes of data has become a critical driver for innovation and decision-making. To a great extent, though, as these data-driven technologies' power grows, so do concerns about data privacy and security and how these selfsame tools are being used. In this article, we will be reviewing some core practices and principles that a data science professional should work on to instill trust and transparency within their work: from data privacy and security to explainable AI.
The Need for Data Privacy and Security
Data privacy and security form the most important contemporary concerns in the data science landscape. The potential impact of mismanagement or improper use could render serious consequences to individuals, organizations, and society in general. One should be very informed about ethical and legal implications of data gathering, storage, and usage when working on data science, and should implement robust safeguards for the protection of privacy and security of data that is being worked on.
Development of Robust Data Governance Frameworks
Effective data governance will be an important part of making data science practices worthy of trust and transparent. This/model will establish clear policies for the management and procedures of data, data classification, access controls, and retention schedules. Thereby, by putting in place a comprehensive framework of data governance, data science teams will ensure responsible and compliant handling of data, mitigating the risks relating to data breaches and unauthorized access.
Protection of Data: Compliance with Regulations
In the contemporary global scenario, professionals relating to data science should be familiar with all the data protection regulations and standards in force that make provisions for the use of personal and sensitive information. This shall include regulations like the General Data Protection Regulation in the European Union, Health Insurance Portability and Accountability Act in the United States, and a host of other industry-specific guidelines. Not only are these regulations legally binding, but adherence to them will also become one of the most important factors in developing trust and upholding the integrity of data science practices.
Embracing Explainable AI for Transparent Decision-Making
As machine learning and artificial intelligence take the lion's share in data-driven decision-making, so grows the need for transparency and interpretability in these systems. Explainable AI—an area of research focused on developing models to provide transparent and understandable explanations for their output—is an important basis for trust and accountability in the application of technologies such as these.
Fostering Interpretability in Machine Learning Models
Connected to the notion of explainable AI is that of interpretability in machine learning models. Model interpretability is important, since by developing intrinsically more interpretable models, one can make sure that data science professionals communicate clearly with stakeholders and end-users about how the model arrived at its predictions or recommendations, enabling informed decision-making and mitigating risks of opaque "black box" algorithms.
Communicating Model Limitations and Uncertainties
One cannot imagine a critical component of trust and transparency in data science practices without effective communication. Data scientists should be clear about the limitations and uncertainties that models carry. They need to explain all assumptions, biases, and probable sources of error that may affect the reliability and validity of the results. This level of transparency manages stakeholder expectations and fosters responsible data science.
Role of Data Scientist Training and Placement in Promoting Ethical Practices
Considering the ever-growing demand for data science professionals who exhibit ethical practices, there couldn't have been any other opportune time for comprehensive training and placement programs. A module in data privacy, security, and ethical AI can help provide aspiring data scientists with a more appropriate base for steering through the complex quagmire of ethical considerations malaise surrounding data-driven decision-making procedures.
Cultivating a Culture of Responsible Data Science
Ultimately, building trust and transparency in data science requires that organizational practices harness a holistic approach reaching beyond the rim of projects or unilateral initiatives. By establishing a culture of responsible data science—the backbone of ethical conduct infused deep into an organization's framework—a data science team can better foster accountability, drive best practice, and engender long-term trust with stakeholders and the public at large.
Future Trends and Considerations
New challenges and considerations in the trust and transparency space will continue in the near future as data science continues to evolve with, for instance, a rising use of federated learning, redistributed forms of processing, algorithmic auditing, mitigation of bias, and addressing ethical implications of a new wave of technologies such as generative AI and reinforcement learning.
Conclusion
Trust and transparency in data science practices form a moral imperative, but it is also a strategic need in a strongly data-driven world. This will help data professionals to ensure that work creates transformative value while securing the highest ethical standards by placing a strong emphasis on data privacy and security, explainable AI, interpretable models, and a culture of responsible data science.
Comprehensive Data Science training and placement programs can assume a very important place in promoting ethical practices given the ever-growing demand for skilled data scientists. It is through such professional programs that aspiring professionals could be equipped with the necessary knowledge and skills to sail through the complex ethical landscape of data science, and thereby play a main role in shaping the future of this dynamic, ever-evolving field.
About the Creator
jinesh vora
Passionate Content Writer & Technology Enthusiast. Currently Working in BIA as a Digital Marketer.
Enjoyed the story? Support the Creator.
Subscribe for free to receive all their stories in your feed. You could also pledge your support or give them a one-off tip, letting them know you appreciate their work.
Comments
There are no comments for this story
Be the first to respond and start the conversation.