Walking Through Online Food Delivery Aggregator Data Analytics
Online Food Delivery Aggregator Data
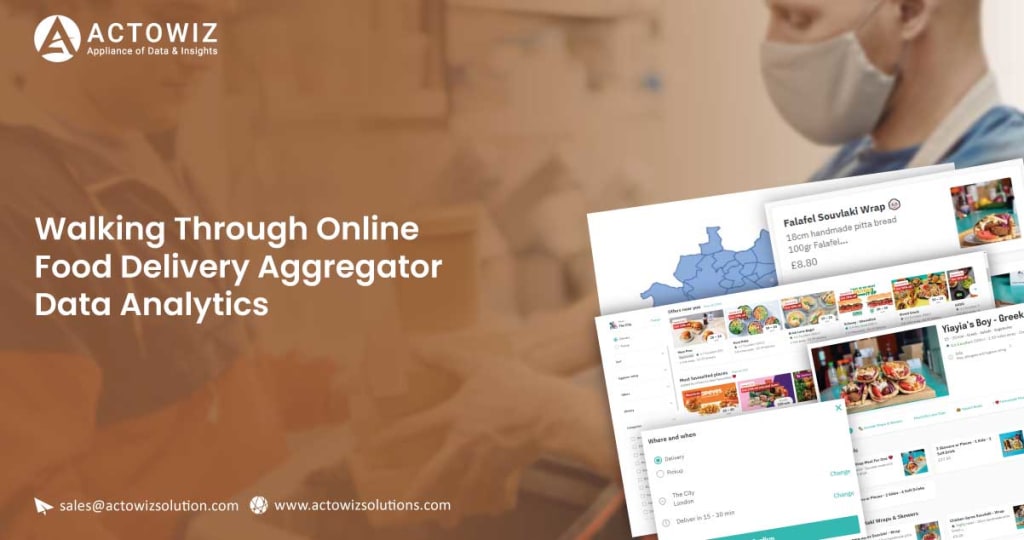
Walking-Through-Online-Food-Delivery-Aggregator-Data-Analytics.jpg
Recently, we shared how to extract data from food delivery websites and process and mold it into a visual map with python. Let's discuss further to resolve a few queries, as shown below.
Recently-we-shared-how-to.jpg
Based on the input address, as shown in the below map, we recognized all the QSRs within the range of delivery. We filtered them into different categories based on delivery fees in different colors. As predicted, we found that the higher the delivery fees of the restaurant, the highest the delivery. As indicated in the map, you can see a circular boundary with orange shading. Beyond that boundary, the delivery fee is greater than 5 USD. But, there are a few points beyond the boundary where the delivery fee is less than 5 USD.
To understand the factors influencing these outliers fees in a better way, we will separate points outside the orange boundary and compare their features to those points inside the boundary. To do so, we will choose the points that create this orange boundary and use them to draw a polygon in the script. Then, we will check the points in the datasets and their location to analyze further.
To-understand-the-factors-influencing.jpg img-2.jpg
We observed the general trend in an outlined area with red shading, where delivery fees increase with the input address distance. But, the points outside the mapped polygon don't follow the pattern (black points are an exception), and we can consider them as outliers. We need to convert these coordinate points for each restaurant into a geometric point to compare the features of these QSRs inside and outside the polygon and then find whether these points are inside the polygon or outside.
We-observed-the-general.jpg We-observed-the-general-2.jpg img-3.jpg
In the dataset, our function determines whether the restaurant is inside the polygon or not, and the last column shows that. To study the characteristics of the restaurants in both categories, we will separate the dataset into 2 groups.
In-the-dataset-our-function.jpg
The most impactful factor on the delivery fees seems to be the distance between the restaurant and the delivery location. We will consider this feature to begin.
The-most-impactful-factor.jpg center.jpg
The majority of restaurants inside the polygon are situated 1 to 3 km from the input address, and their delivery fees range from 0 to 7 USD. Apart from this, restaurants located between the 4 to 7 km range have a probability of delivery charges below 5 USD.
From the analyzed data, we observed that most of the restaurants located outside the polygon have zero delivery fees, which means the delivery distance needed to be more accurate. We saw the delivery fees of 7 USD for the restaurants within the polygon. This reflects that restaurants outside the created polygon have considerably fewer delivery fees as compared to the inside restaurants. Moreover, analyzing the potential impact on delivery fees, we selected a group of columns with our assumptions and the output of the correlation matrix measuring the strength of relationships of values.
df.corr()['deliveryFeeDefault'].sort_values(ascending=False)[2:]
As per the correlation matrix, is delivery by scoober is the main factor with a strong correlation with the delivery fees. There is a possibility that other factors could have a weaker correlation because of the existence of outliers. Therefore, we will further investigate by plotting the data.
4234.jpg As-per-the-correlation-matrix.jpg As-per-the-correlation-matrix-2.jpg img-4.jpg
After plotting the selected data column groups, we can see all the restaurants in the polygon plotting. The columns pricerange, and isnew have the same distribution. But no restaurant outside the polygon used scoober delivery, and the sponsored restaurant distribution looks more balanced. The least order value also varied more in this portion considerably.
Let us check the restaurant distribution that uses scoober delivery, where we will plot two plots parallelly using the below function. This will help us to compare both groups easily.
Let-us-check-the-restaurant.jpg Let-us-check-the-restaurant-2.jpg img-5.jpg
The boolean value shows whether a restaurant is using scoober delivery or not likely plays a major role in delivery fees of restaurants, as you can see in the red shared range in our study earlier. The reason is that restaurants are making agreements with lieferando to use scoober delivery services, where scoober will work as a food delivery aggregator. To further investigate the relation between delivery fees and scoober, we can categorize the data for the restaurants associated with scoober delivery and study the relation between delivery fees and other factors.
The-boolean-value-shows-whether.jpg img-6.jpg
Here, the distance is related to delivery fees on a positive note, where the output has grown from .15 to .75
It is worth noting that the order's base value has NaN, meaning the order value is constant or missions. Let's analyze these restaurants again for the case where lieferando doesn't use scoober delivery for food shipment, considering that these restaurants are using their delivery platforms.
It-is-worth-noting-that-the.jpg Last.jpg
Here, we couldn't see any improvement with the distance remaining constant at 0.20, and the minimum order value was 0.4, way higher than the distance.
When our team plotted the least order values for Huston-based restaurants to check whether they use scoober for delivery service, we got the results below.
img-7.jpg
It looks like lienferando has a base value for orders of 8 USD for each restaurant that offers scoober delivery service. It may be because of the agreement between scoober delivery and restaurants. It is the same as another food aggregator Wolt as they also have a base order value of 8 USD for every restaurant.
Our team created histograms to compare selected columns on the condition of whether restaurants used scoober delivery or not. This helps to quickly overview the notable variations between the two groups we created for analysis.
img-8.jpg img-9.jpg
Summary Of The Analysis
We found that around 25 percent of existing restaurants don't offer scoober delivery with their order value between 8 to 45 USD, and low delivery fees despite the delivery distance. Whereas, the rest of the restaurants offer scoober delivery, with minimum order value being 8 USD and higher delivery fees.
Do you want to do the food delivery platform analysis for your restaurant business using our mobile app scraping and web scraping services to work on food delivery data analytics? Do contact us at Actowiz Solutions, and we will revert you instantly.
About the Creator
actowizsolutions
We are the leading web data scraping solution company big-scale public data assembly, driven by innovations and higher business standards.Actowiz has created enterprise-grade solutions to empower organizations to deliver the correct .
.
Comments
There are no comments for this story
Be the first to respond and start the conversation.