Skin Cancer & Computer Vision - A New Sense?
Computer vision in cancer requires reliable, valid data to truly see.
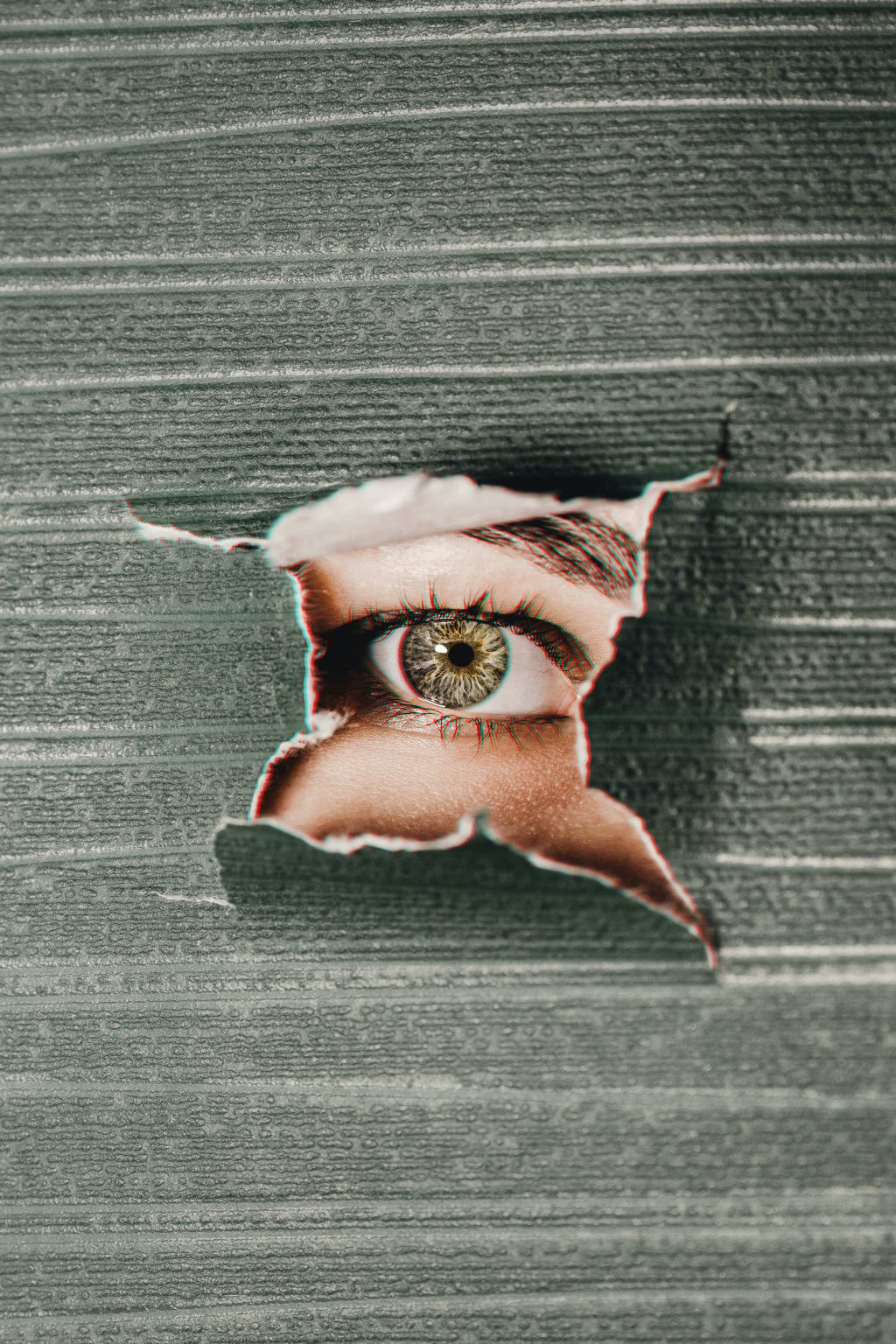
This article was previously posted on Dr. James Goydos' Medium.
When we think of vision, we may first think of the most obvious association: the eyes. Our sense of vision is perhaps one of our most significant, and it is certainly the more studied sense in comparison to taste, touch, smell, hearing, and others such as proprioception. While its significance in context may be debatable, it is one of the first modalities with which many humans consciously engage with the environment.
Vision is an important sense to many species, enabling access to visual information that can be used to enhanced decision making. The utility of vision, however, extends beyond humans, and into new technological advancement with computer vision. Computer vision is not too unlike human vision. Simply put, computer vision is a scientific field that seeks to understand human visual perception to automate visual tasks with the use of technology.
Computer vision can transform cancer diagnosis, treatment, and prognosis.
Why is this important? Computer vision has a wide range of applications from the automotive industry to healthcare and more. Its use can be seen in the technology of autonomous vehicles, for example, helping a car to 'see' and make driving decisions such as when to avoid an obstacle.
The applications of computer vision to healthcare are multi-faceted and evolving daily. Google's Artificial Intelligence (AI) famously made headlines regarding their detection of breast cancer with an AI algorithm, when its accuracy of breast cancer detection was reported to outpace that of doctors.
Computer vision's applications in the detection of cancer is an interesting one, given the potential to transform diagnosis, treatment, and prognosis significantly. There are several potential use cases for computer vision regarding the dynamics of cancer; however, of particular interest is its relevance to skin cancer and soft tissue tumors.
With increased access to data via open data portals, it has never been easier to get datasets for a myriad of uses. This is important. Understanding cancer and increasing the accuracy of skin cancer detection require valid, reliable data. And this often means collaborating. Sharing data and resources. Cancer research is a collective effort and requires collective solutions. The dynamics of cancer may be different in various populations. Therefore, it is important that we can access data from differing populations.
Increasing access to open data has the potential to improve a myriad of industries, and healthcare is no exception. Increased access to valuable data can help improve technological innovations such as machine learning models in medicine. Reliabe datasets can help improve medical algorithms and can also improve clinical trials. These are a few of the many benefits associated with open access to information.
"Computer vision in cancer requires reliable, valid data to truly see".
In the absence of reliable valid data, one cannot rely on any of the outputs of medical algorithms. As they say, 'Garbage in, garbage out'. If a medical application does not have enough data to conduct its job--if its inputs are unreliable--it's outputs will be, too.
But more data doesn't necessarily mean better. Quality and quantity are both important considerations when it comes to valuable data. One does not want too little data to conduct their task, as they can run the risk of overfitting, or simply not giving a representative outlook of the data at hand. Scientific rigor is not just for a doctor's laboratory, but applies in data science, too. The value of quality data should not be overlooked. Data should be representative, diverse, and checked for quality prior to be applied in a medical context, or any domain.
As technology continues to evolve, it is important that our understanding evolves in tandem. Nothing operates inside a vacuum, including science. We must delve deep into our potential biases and our hypotheses, lest our data and subsequent findings become unreliable. As they say in computer science, 'garbage in, garbage out'. If we fail to consider these foundations, the buildings which we create in the field of computer vision may be quick to crumble.
About Dr. James Goydos
Dr. James Goydos is an expert in melanoma research and specialist in surgical oncology with an M.D. from Rutgers University. With over 20 years of experience as a Professor, Surgeon, and Clinical Trial Lead, he is a leading expert in his field.
Subscribe to James Goydos’ newsletter. Follow me on Good Men Project, Newsbreak, Hubpages, Loop, Medium, Instagram, Facebook,YouTube, Medika Life, Doximity, Github, Kaggle, Vocal, LinkedIn,Pinterest, Wordpress, YourStack, Carrd, and Twitter!
About the Creator
James Goydos, MD
James Goydos MD - Doctor, surgeon & expert on skin cancer. M.D. from Rutgers. Experience as a Professor of Surgery, Surgical Oncologist, & clinical trial leader. Writing on cancer, detction with camera / computer vision and healthcare.
Comments
There are no comments for this story
Be the first to respond and start the conversation.