AI: An Ace of Technology for A Healthier Healthcare System
AI has potential to power up healthcare system. From AI for medical diagnosis to surgeries, this technology can spruce up all spheres of healthcare industry.
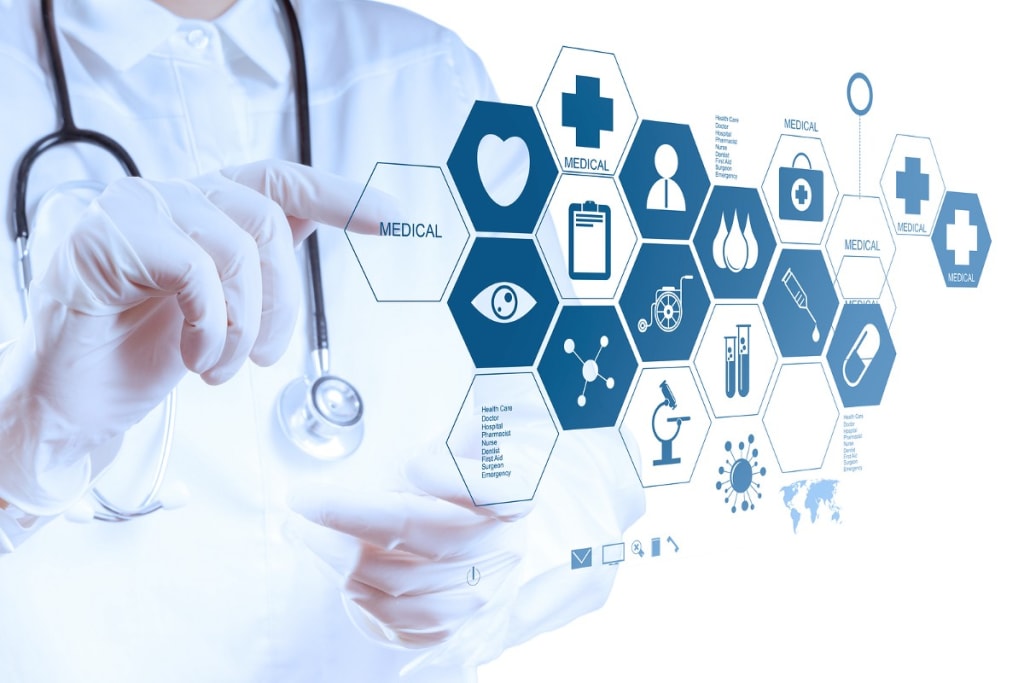
Artificial Intelligence (AI) is all the buzz around as it goes about automation and its integration into life-led systems. It’s all for the sake of ease and improved efficiency. The world, as we see it, stands to benefit greatly from AI implementation. While the adoption of AI has already been widespread in certain industries, the healthcare industry yet remains to keep up with the course — the course of the fast-changing symmetry of AI penetration into clinical practices and procedures.
The scope of deploying artificial intelligence in medicine, treatment, and diagnostic procedures is broad, and we have testimonies of AI developments happening around healthcare practices. However, the progress of data annotation & training data development processes — which run at the backend and which make the real bid for fast-paced AI-enabled healthcare models — are worth watching over.
Possibilities AI Holds for Better Healthcare System
Needless to say, AI has the potential to power up healthcare facilities and treatment procedures in several ways with automation. From diagnostics to treatment to surgical procedure, AI, in all honesty, shows its significance to spruce up almost every sphere of the healthcare industry — as we see it being deployed in different facets of clinical practices.
Harnessing AI for medical diagnosis and clinical treatment procedures can bring a breeze to healthcare practitioners allowing them to focus more on patient care & wellness. The healthcare tools and processes backed by artificial intelligence, natural language processing (NLP), and image & predictive analytics based on machine learning can help healthcare delivery systems take a turn for the better with more accuracy and acceleration.
Thus, the possibilities of AI for better healthcare practices are beyond the limit. However, the backend medical data annotation and image labeling processes — which are the linchpins for developing quality training data for AI-based healthcare care models, need to be beefed up.
Need of Quality Training Data for Developing AI Healthcare Systems
“AI will not replace doctors but instead will augment them, enabling physicians to practice better medicine with better accuracy and increased efficiency” — the revelations made by one of the renowned Scottish scientific surgeons Benjamin Bell in a time of underdeveloped AI era makes a true manifest about the expanding role of artificial intelligence for better clinical and healthcare practices. But the possibilities that AI holds for healthier healthcare practices go parallelly in sync with the pace of medical data annotation processes that operate in the backyard.
Errors or inaccuracies, which might pop up out of nowhere when working with AI-operated medical systems, are intolerable. Quality training data is the grist for the mill when it comes to developing flawless AI-powered means and mechanisms for implementing in medical practices. This being the case, finding the right partner for medical annotation is a matter of significance.
CogitoTech, Analytics.AI, and to name a few more have their repute on display for quality data labeling — their role is disruptive when it comes to contributing to the development of ready-to-integrate AI models into different vertices of healthcare, i.e., from diagnosis to surgical procedures to hospital management.
Applying AI to Healthcare — Types & Tacks
Healthcare models developed with accurately integrated AI algorithms has the potential to supersede human expertise and it’s agreeable seeing the potential of AI. However, AI will take many years from now to replace humans for a majority of medical processes despite its vast applications in healthcare practices for the time being. With all that being said, AI still has a substantial participation in maneuvering healthcare practices and the types and tacks spelled out herein reveal it all.
In healthcare, the most common application of traditional machine learning is precision medicine – predicting what treatment protocols are likely to succeed on a patient based on various patient attributes and the treatment context.2 The great majority of machine learning and precision medicine applications require a training dataset for which the outcome variable (eg onset of disease) is known; this is called supervised learning.
Here are the five major applications of AI in the healthcare industry:
Natural Language Processing for Clinical Documentation
The potential of NLP can be brought into use in healthcare to create, comprehend, and categorize clinical documentation and research publications. A conversational AI system can help practitioners analyze unstructured clinical notes about patients and prepare reports (e.g. on radiology exams), and transcribe patient interactions in line with the course of treatment.
Robotics for Intensive Surgical Procedures
Robots are the machines of the future. There will be the time when will see robots standing on every front line handling all major tasks from repositioning to lifting to assembling to organizing everything that is handed over to them.
The Healthcare industry also has a fair share of benefits to take from machine learning models as the AI-embedded systems can bring significant aid to surgical procedures, particularly the ones that are too intricate to handle manually, e.g., gynecologic, prostate, and head & neck surgeries.
AI Application in Diagnostic and Treatment Practice
Tech firms and startups are working assiduously on developing AI-based solutions for better diagnostic and treatment practices. From major tech conglomerates to budding AI/ML start-ups are collaborating to build clinic-specific prediction models that can help healthcare providers with the right sense for high-risk clinical conditions. Google, the American tech giant, is working with a multitude of health delivery networks to power up diagnostic and treatment processes with AI-integrated healthcare solutions.
AI for Better Patient Engagement
Deeper involvement by patients can result in better health outcomes. AI-based capabilities can be effective in personalizing and contextualizing care with improved patient engagement. Technology, in a broader sense, has gradually become a helping hand for the 21st-century generation as it is instrumental to keeping the routined human life in sync with other obligations.
AI-embedded tools & applications can work as a substantial means to keep a patient's routine in sync with the clinical procedures just in case he fails to follow the treatment plan in compliance with the behavioral change or forgets to fill prescriptions or misses scheduling follow-up appointments.
AI for Drug Discovery
Unleashing the untapped potential of AI has a long list of opportunities for improved drug discovery processes which stretch from preclinical studies to compound screening to clinical trials and validation. AI can enhance drug development by removing the limitations imposed by outdated technologies which make the course of drug discovery practices a labor-exhausting, time-consuming, and cost-intensive affair.
The data sets used for drug development at pharmaceutical companies can involve millions of compounds, and traditional machine learning tools cannot cope with such data. By utilizing AI, drug targets and drug structure designs can be validated quicker with fast identification of lead compounds in the process. AI can assist inclusively in the drug design process when it comes to developing personalized medicines, managing the clinical data generated, and applying it for next-generation drug development.
Final Thought
Artificial intelligence is indeed the ace of technology that holds the command of healthier healthcare practices. The use of AI systems in healthcare is not expected to replace clinicians across the board, but rather to augment their efforts.
As time progresses, healthcare experts will likely leave intensive clinical and surgical chores to be managed through AI while drawing their focus more on human attributes for a personalized healthcare experience.
About the Creator
Matthew McMullen
11+ Years Experience in machine learning and AI for collecting and providing the training data sets required for ML and AI development with quality testing and accuracy. Equipped with additional qualification in machine learning.
Comments
There are no comments for this story
Be the first to respond and start the conversation.