Separating Artificial Intelligence and Deep Learning
Or Is AI Divorcing Deep Learning?
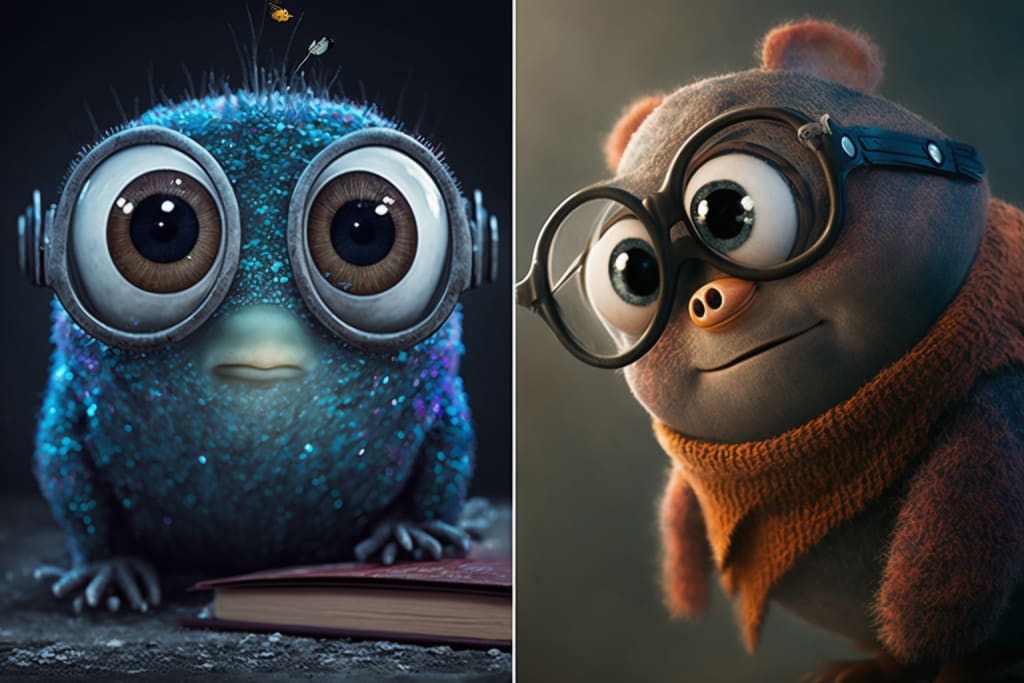
As technology advances, Artificial Intelligence (AI) and Deep Learning (DL) are becoming increasingly intertwined. However, it is important to understand the differences between the two concepts in order to better understand how they can be integrated into our daily lives. This article will explore the distinctions between AI and DL, as well as how they can be used together to create more powerful technologies.
This article is sponsored by AI-Info.org
Explaining AI & Deep Learning
Deep learning is a subset of artificial intelligence that uses neural networks to learn from large amounts of data and build complex models. It is based on the idea that machines can use these models to make decisions more accurately than humans. Deep learning algorithms draw from multiple layers of neural networks, which are a series of interconnected nodes that feed information forward and backward for decision-making. Compared to traditional AI algorithms, deep learning has significantly improved accuracy and efficiency in tasks such as object detection, natural language processing, and speech recognition.
The key difference between artificial intelligence (AI) and deep learning lies in the approach used for problem solving. AI relies on explicit programming whereas deep learning uses an iterative process where computers are able to learn through experience by analyzing data sets without being explicitly programmed with rules or instructions. This allows deep learning algorithms to become increasingly accurate over time as they continue to be exposed to new data sets. In contrast, AI solutions require manual programming before they can be deployed into production systems; thus requiring more resources upfront but providing greater control over their behavior in specific scenarios.
AI: Definition & Examples
AI, or Artificial Intelligence, is a broad term that refers to the ability of machines to learn and act like humans. It involves a variety of techniques and technologies designed to empower computers with human-like abilities such as recognizing patterns, understanding complex problems, and making decisions. AI relies on neural networks to process data and come up with solutions.
A subset of AI is deep learning, which involves using large datasets of training data in order to train artificial neural networks that can recognize patterns in information without explicit programming. Deep learning algorithms are used for applications including computer vision, natural language processing, speech recognition, robotics and more.
Examples of AI include self-driving cars which use sensors to detect objects around them while driving; facial recognition software which uses pattern recognition algorithms to identify people; virtual assistants like Siri or Alexa which can respond based on voice commands; medical diagnosis tools which use AI technology to diagnose patients; and automated home security systems which monitor homes using cameras and motion detectors.
Deep Learning: Definition & Examples
Deep learning is a subset of artificial intelligence (AI) that uses algorithms to imitate the brain’s structure and functioning. It enables machines to ‘learn’ from input data, identify patterns and make decisions with minimal human intervention. Deep Learning relies heavily on neural networks - layers of interconnected nodes - which allow computers to process complex tasks more efficiently than traditional algorithms.
Examples of deep learning applications include computer vision, natural language processing, speech recognition and machine translation. For instance, deep learning can be used for object recognition in an image or video, text-to-speech conversion or autonomous driving. It can also provide insights into datasets by finding meaningful correlations between variables that humans would be unable to detect or analyze quickly enough. By using deep learning models like convolutional neural networks (CNNs), researchers are able to leverage large amounts of unlabeled data and achieve better results with less effort than traditional methods such as feature engineering and manual labelling.
Differences Between AI and DL
Artificial intelligence (AI) is an umbrella term for computer systems and algorithms that can solve complex tasks, such as making decisions and recognizing patterns. AI is not a specific algorithm or program, but rather it's a set of techniques and approaches that can be used to build intelligent machines. Deep learning (DL), on the other hand, is a subset of machine learning which uses neural networks to learn from large amounts of data. It can be used for solving complex problems such as image recognition and natural language processing.
The main difference between AI and DL lies in their approach towards problem-solving: while AI typically relies on explicit programming, DL requires no prior knowledge or instruction. Instead, it uses methods like backpropagation and optimization algorithms to learn from data sets with millions of examples. This allows DL models to generate more accurate results by taking into account all available data points rather than relying on predetermined rules like in AI. Additionally, while AI focuses only on performance metrics such as accuracy rate or time-efficiency, deep learning also takes into account features such as interpretability or explainability – explaining why certain decisions have been made using data-driven techniques instead of programmed logic.
Challenges in Separating AI and DL
One of the major challenges in separating artificial intelligence (AI) and deep learning (DL) is data labeling. For example, when training a machine learning algorithm, the data must be labeled – that is, it needs to be identified in terms of what it represents. This label helps to identify patterns and trends within the data set which can then help inform decisions on how to use AI or DL for a particular purpose. However, labeling data is often difficult as there may not be any existing labels for certain types of datasets, such as images or audio recordings. Additionally, any existing labels may not always reflect real-world concepts accurately which further complicates decision-making from an AI/DL perspective.
Another challenge related to separating AI and DL is the lack of an appropriate feature set for modeling complex problems. DL models require large amounts of labeled data in order to learn the underlying patterns within the dataset that can help generate accurate predictions; however, when dealing with more sophisticated problems such as predicting stock prices or customer behavior, finding sufficient labeled data can prove difficult if not impossible due to its complexity. As such, this makes it harder for models built using deep learning techniques to capture these nuances which could lead them to making inaccurate predictions.
Applications of AI and DL
AI and DL both have applications in many industries, but they are used differently. AI is a broad umbrella term that describes the ability of machines to independently perform tasks that would typically require human intelligence. AI can be used for functions such as decision making, natural language processing, facial recognition, and other cognitive activities. DL is a specific type of AI which uses algorithms to process data and extract patterns from it. It can be used for speech recognition, image processing, object tracking or detection, among other things.
In the healthcare industry, AI and DL can be used to diagnose diseases faster and more accurately than traditional methods. For example, medical images like X-rays or MRI scans can be analyzed by computer vision systems powered by DL algorithms to detect signs of cancer or heart conditions more quickly than manual analysis alone. In addition, voice assistants powered by natural language processing (NLP) algorithms enable doctors to access patient information quickly while providing them with advice on treatments or medications based on their needs.
In finance and banking fields AI and DL are also proving useful in fraud detection and money laundering prevention efforts because they allow financial institutions to analyze large amounts of customer data faster than humans ever could before. Similarly, automated trading platforms—powered by AI technology—allow investors to make stock trades without having deep knowledge about the markets because decisions are made algorithmically using historical market data as input.
Current Limitations
One of the current limitations of artificial intelligence and deep learning is their inability to explain why they make the decisions they do. While AI and deep learning algorithms are becoming increasingly accurate, there is still no way for experts to understand the reasoning behind those decisions. This means that any errors or biases present in the training data could be perpetuated by the algorithm's output. Furthermore, it raises questions about accountability when AI-powered systems make mistakes or display bias.
Another limitation is computing power. AI and deep learning algorithms require a significant amount of processing time, as well as powerful hardware like GPUs to run effectively. This can limit where these technologies can be implemented due to cost constraints, making them difficult to adopt in certain areas such as healthcare or education where budgets are often limited. Additionally, many existing AI solutions rely on large datasets which can also increase costs significantly.
Conclusion
"The difference between Artificial Intelligence and Deep Learning is clear: Artificial Intelligence is the ability to think, while Deep Learning is the ability to understand."
In conclusion, Artificial Intelligence and Deep Learning are two distinct fields of study, although they are related. AI is a broad term that encompasses a wide range of technologies from computer vision to natural language processing and robotics. DL is focused on the use of neural networks to "learn" from large datasets in order to classify or make predictions. AI can be used in many different applications such as self-driving cars, facial recognition systems, and automated customer service bots. However, when it comes to deep learning, it has become the preferred approach for many machine learning tasks due to its ability to learn patterns from data sets more accurately than traditional methods. While both AI and DL have their strengths and weaknesses, they complement each other in today's world of computing technology by allowing us to develop more sophisticated solutions faster than ever before.
About the Creator
Patrick Dihr
I'm a AI enthusiast and interested in all that the future might bring. But I am definitely not blindly relying on AI and that's why I also ask critical questions. The earlier I use the tools the better I am prepared for what is comming.
Comments (1)
very informative content. what's your take on Dal-e ?