Machine Learning in Healthcare: Opportunities and Challenges
Machine Learning
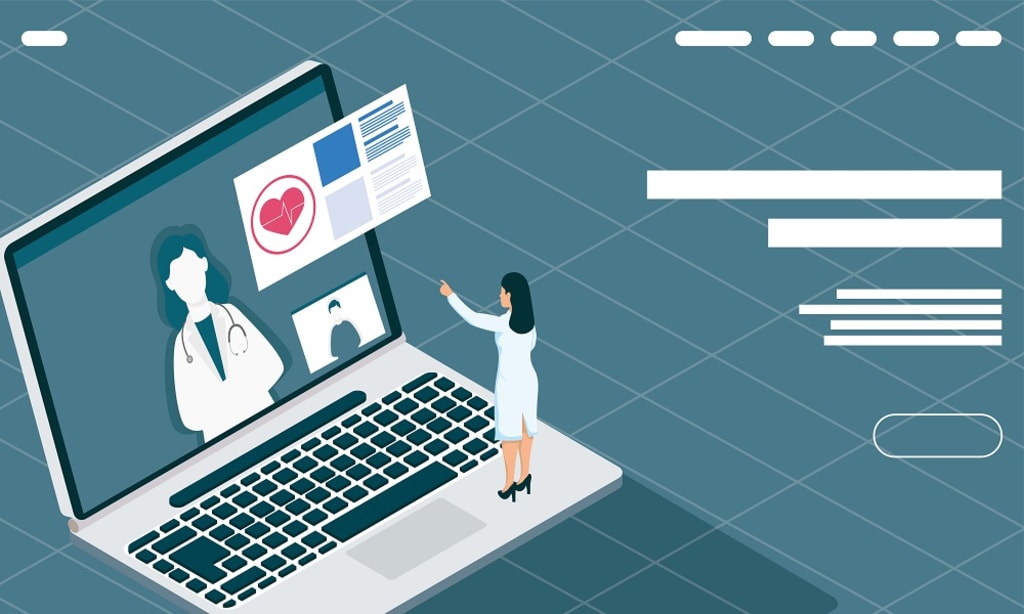
Machine learning (ML) has revolutionized various industries, including healthcare. With its ability to identify patterns and make predictions from vast amounts of data, ML has the potential to improve patient outcomes, reduce costs, and enhance the overall quality of healthcare. However, as with any new technology, there are also challenges and potential risks to be addressed. In this article, we will explore the opportunities and challenges of using machine learning in healthcare.
Introduction
The healthcare industry has been generating vast amounts of data for years, from electronic health records (EHRs) to medical imaging. However, making sense of this data has always been a challenge. This is where machine learning comes in. ML algorithms can analyze and learn from large volumes of data, enabling healthcare professionals to make more informed decisions and improve patient outcomes.
What is Machine Learning?
Machine learning is a subfield of artificial intelligence (AI) that focuses on developing algorithms that can learn from and make predictions on data. These algorithms can identify patterns and make predictions without being explicitly programmed to do so.
Applications of Machine Learning in Healthcare
Machine learning has numerous applications in healthcare, including:
- Medical image analysis
- Electronic health record analysis
- Drug discovery
- Clinical decision support
- Predictive analytics
- Personalized medicine
Opportunities of Machine Learning in Healthcare
Improved Diagnosis and Treatment
ML algorithms can analyze medical images and provide insights that may not be immediately apparent to human physicians. For example, a ML algorithm can analyze a mammogram and detect subtle changes that may indicate breast cancer. ML can also analyze EHR data and identify patients at risk of developing certain conditions.
Personalized Medicine
ML can help tailor treatment plans to individual patients based on their genetic makeup, medical history, and other factors. This can improve treatment outcomes and reduce the risk of adverse reactions to medication.
Predictive Analytics
ML can analyze large datasets to identify patterns and make predictions about future outcomes. For example, ML can analyze EHR data to predict which patients are at risk of readmission or developing complications after surgery.
Cost Reduction
ML can help reduce healthcare costs by identifying inefficiencies and areas where resources can be better allocated. For example, ML can analyze claims data to identify fraudulent claims or overbilling.
Challenges of Machine Learning in Healthcare
Data Quality and Quantity
ML algorithms require large volumes of high-quality data to learn and make accurate predictions. However, healthcare data is often incomplete, inconsistent, and scattered across different systems, making it challenging to use for ML purposes.
Bias and Ethical Concerns
ML algorithms can be biased, which can lead to unfair or discriminatory treatment of certain patient groups. Additionally, there are ethical concerns around the use of ML in healthcare, particularly around issues such as data privacy and consent.
Integration with Existing Systems
Integrating ML algorithms with existing healthcare systems can be challenging. Many healthcare systems use legacy software and hardware, which may not be compatible with newer ML technologies.
Regulatory and Legal Issues
There are numerous regulatory and legal issues to consider when using ML in healthcare. For example, there are concerns around the accuracy and reliability of ML algorithms, as well as issues around liability and accountability in the event of errors or adverse outcomes.
Conclusion
Machine learning has enormous potential to transform healthcare by improving diagnosis, treatment, and overall patient outcomes. However, there are also significant challenges that must be addressed, including data quality and quantity, bias and ethical concerns, integration with existing systems, and regulatory and legal issues.
To fully realize the potential of machine learning in healthcare, stakeholders must work together to address these challenges and ensure that ML is used ethically and responsibly. This includes investing in high-quality data infrastructure, ensuring algorithmic transparency and accountability, and promoting a culture of ethical AI in healthcare.
As we continue to develop and refine ML algorithms for healthcare, it is important to prioritize patient safety and privacy. By doing so, we can unlock the full potential of this powerful technology to improve the lives of millions of patients around the world.
About the Creator
Rehana Atar
SEO Expert and content writer
Enjoyed the story? Support the Creator.
Subscribe for free to receive all their stories in your feed. You could also pledge your support or give them a one-off tip, letting them know you appreciate their work.
Comments
There are no comments for this story
Be the first to respond and start the conversation.