Empowering BI Tools for Machine Learning Success Through Data Transformation
Machine Learning Success
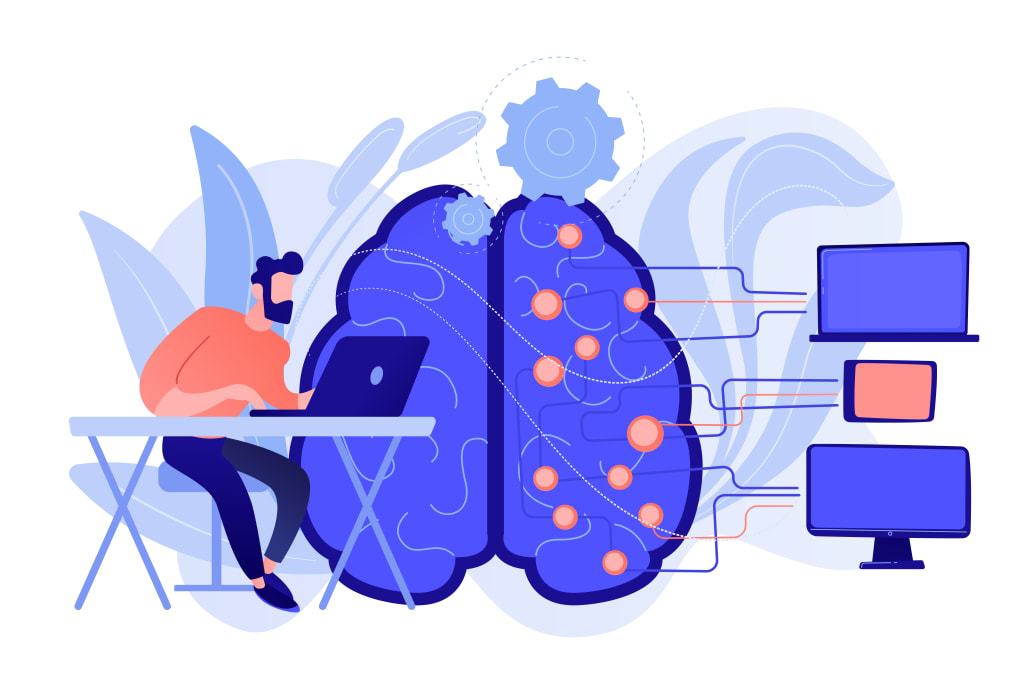
Table of Contents:
1. Introduction: The Crucial Role of Data Transformation in Machine Learning
2. Understanding the Machine Learning Data Pipeline: Where BI Tools Fit
3. Techniques in Data Profiling for Identifying Data Quality Issues
4. Dealing with Missing Values and Outliers: Leveraging BI Tools in Data Cleaning
5. Encoding of Categorical Features: Transferring Text into Numbers with BI
6. Derived Features: How to Combine or Transform the Data with BI
7. Feature Scaling and Normalization: Ways of Preparing the Data before Model Training with BI
8. Contribution of Best Data Science Courses in Chennai in Mastering BI-Powered Data Transformation
9. Feature Importance: Assessment of the Impact on Model Performance
10. Feature Iteration and Refining: An Ongoing Process in BI-Powered Enhancement
11. Case Studies: Effective Real-World Strategies of BI-Driven Data Transformation
12. Conclusion: Embracing Business Intelligence Tools for an Accurate and Reliable Machine Learning Model
Introduction
In machine learning, data transformation is a big determinant factor.
Few things matter more in Machine Learning than the quality and relevance of input data in general or more specifically, accuracy of predictive models. But the source data often comes with a host of issues: missing values, inconsistent formatting, and irrelevant features— all of which can put a dent in how effective machine learning algorithms could turns out to be.
This is where data transformation steps in—availing Business Intelligence tools with preprocesses and engineering input for machine learning projects. Proper selection, cleaning, and mapping of raw data into a format more suitable and informative with respect to machine learning algorithms will help the data scientist significantly improve model accuracy and generalization.
This will be a comprehensive guide on how BI tools can be used in feature engineering and cleaning of data in machine learning projects, important techniques, and best practices involved, and see how the best data science courses in Chennai can help one master this very important skill.
Understanding the Machine Learning Data Pipeline: Where BI Tools Fit In
Before getting into specifics related to data transformation powered by BI, it is relevant to frame this within the context of a more overarching workflow in machine learning. Typically, this pipeline of machine learning consists of a few chief stages: data collection, preprocessing, feature engineering, model training, evaluation, and finally, deployment.
Data transformation lying at the heart of this pipeline will act as the bridge between raw data and model performance. This is very important, especially when BI tools are used to clean, transform, and engineer input data that goes into machine learning models. Data scientists will be using high-quality input information that enables machine learning models to best capture the underlying patterns in data and predict accurately.
Most of the best data science courses in Chennai will give students an overview of the machine learning pipeline and furnish them with a very strong conceptual basis for how transformation fits in this wider workflow. By mastering this context, mostly one can grow a much more holistic and strategic approach toward building effective machine learning solutions.
Identifying Data Quality Issues: Techniques for Data Profiling
One of the major challenges in the process of data transformation is to be able to identify and deal with data quality issues that may affect machine learning models. Data profiling techniques enable data exploration and statistical analysis, helping data scientists find probable problems within their datasets, including missing values and outliers and inconsistencies.
This kind of data profiling functionality generally stretches across most BI tools within a rather wide range, offering the ability to rapidly understand the structure, content, and quality of their data. With this kind of functionality put in place, data scientists will be better positioned in a way that assures meaningful insights to be derived from such datasets about characteristics and be able to pinpoint the improvement areas, thus focusing efforts toward data transformation.
The best data science courses in Chennai would often include data profiling techniques in the context of these BI tools, thereby helping students exercise these methods on real-world datasets. Mastering these skills will ensure that students' data transformation efforts are focused on the most critical issues, thereby improving the overall quality and reliability of the machine-learning inputs.
Missing Values and Outliers: How BI Tools Can Help in the Cleaning of a Dataset
This is one of the most common data quality issues in machine learning projects: missing values, when not repaired, and outliers can have serious consequences on the performance of predictive models. Several techniques in business intelligence tools provide data cleaning facilities for the treatment of such issues, including data scientists, specifically imputation, removal, and robust scaling.
BI tools can be used to clean up these common pitfalls in their datasets and provide a more close-to-reality representation of the data. These will increase the accuracy and reliability of results obtained from machine learning modeling techniques. The best data science courses in Chennai often cover data cleaning techniques in the context of BI tools, whereby students get hands-on training on these methods with real-world datasets.
Encoding Categorical Features: Transforming Text into Numbers with BI
Many real-world datasets have categorical features, which are processable by machines; therefore, some other techniques of data transformation are applied to enable the models to digest these categorical variables in numerical format.
These types of BI tools usually integrate a set of encoding facilities, such as one-hot encoding, which simply creates a binary column for each unique category; label encoding; and target encoding. Equipped with these BI-powered encoding techniques, a data scientist can ensure that their machine learning model is able to effectively harness these categorical features to improve overall performance and accuracy.
The best data science courses in Chennai would most probably teach these methods of encoding with respect to BI tools and give the student an idea as to when to use which of the above techniques and how to deal with high cardinality categorical features (those with too many unique values).
Creating Derived Features: Combining and Transforming Data with BI
Apart from cleaning and encoding the raw data, data transformation also includes the creation of new derived features that will be able to provide more information to machine learning models. Many times, BI tools allow for several data transformations, such as combining columns using arithmetic operations, applying domain-specific transformations, or engineering features using expert-domain knowledge.
Apart from that, a data scientist can also use BI tools in the process of creating derived features to uncover deep insights and patterns in datasets, thereby allowing the machine learning models to make predictions more accurately and informatively. A good many of the best data science courses in Chennai will encourage creative approaches toward feature engineering by teaching students when to identify opportunities for derived features and how to measure the impact on model performance.
Scaling and Normalizing Features: Getting Ready for Model Training with BI
If we go ahead to feed these engineered features into a machine learning model without ensuring features are on the same scale and distribution, this may result in very serious implications. Most machine learning algorithms are sensitive to the scale of the input features, such as linear regression and neural networks, which perform poorly if there is huge variation in the values for the features.
BI tools usually provide for scaling and normalizing data, like standardization—that is, subtracting the mean and dividing by standard deviation—and min-max scaling, where everything gets scaled into a certain range usually from 0 to 1. Powered by these techniques of BI, a data scientist has to be sure that a trained machine learning model is on an even playing field. In other words, he is trying to focus on the most informative features, where machines are not biased by differences in scale.
These data scaling and normalization techniques usually form part of the best data science courses in Chennai, in relation to BI tools. Students will have an opportunity to get hands-on experience applying these methods in their transformation pipeline.
How Best Data Science Courses in Chennai Can Help One Master BI-Powered Data Transformation
The best data science courses in Chennai can be of great benefit to aspiring and experienced data scientists to help them master the tinkering art of BI-powered data transformation. The curriculum brings together the theoretical basis of learning with practical applications and nuances specific to industries involved in leveraging the power of BI tools for feature engineering and data cleaning activities within machine learning projects.
It is such a set of data science courses in Chennai that make up the experiential learning environment whereby students acquire a real understanding of the BI tools and their contribution to machine learning models' performance, right from comprehending how they operate to being able to steer them. Students can be assured and fully equipped with everything needed to shift the challenging, dynamic landscape of data transformation and effectively provide relevant machine-learning solutions to their respective organizations.
Evaluating Feature Importance: How It Impacts Model Performance Measurements
It will be necessary to gauge the importance and contribution of features so that data transformation efforts have an impact on model performance. BI tools often provide a wide palette of feature importance analysis techniques at one's disposal, including measurements of changes in model performance by removing or permutation of a feature, calculation of the correlation of each feature with the target variable, or analysis of coefficients or weights obtained for each feature by the machine learning model.
Knowing what features of the model drive its predictions and knowing the ones that do not is very useful. The data scientist would then tune efforts around data transformation, putting more effort into the most impacting features and possibly dropping or transforming less relevant features. The best data science course in Chennai often covers in-house the techniques for evaluating feature importance with BI tools so that students gain practical experience in applying these methods for their machine learning projects.
Iterating and Refining Features: Levels of Improvement Continue with BI
Transformation of data is not a one-time affair. Rather, it is an iterative process of improvement throughout the workflow of machine learning. During the training and evaluation of models, data scientists have to constantly look at the performance of their transformed features for further refinement and optimization.
This may include new data transformation techniques or other ways of combining features, or it could be another way of encoding and scaling. By working in an iterative way and continuing to update the efforts of data transformation, a data scientist can ensure his machine learning models are always running at the highest level of performance.
Consequently, the best data science course in Chennai would usually emphasize the need for iteration and continuous improvement in data transformation. Students are equipped with ways to structure the experimentation and track the impact of their changes on the accuracy of the model.
Case Studies: Successful BI-Driven Strategies of Data Transformation
Such scenarios can be understood better by going through some case studies of practical applications and also by considering the benefits accruing from BI-powered data transformation in different industries. Those range from domain-specific feature engineering that increases the accuracy of fraud detection models within financial services to including derived features built with domain experts for predictive maintenance within manufacturing. There are several use cases in which effective strategies around data transformation have helped organizations realize significant performance gains.
These case studies are often part of some of the best data science courses in Chennai, which give a deeper drill-down into the challenges, best practices, and lessons learned from various organizations that have successfully undertaken BI-driven data transformation. Students can learn numerous valuable insights from such case studies and even get excellent ideas to pursue for their machine-learning projects or data transformation efforts.
Conclusion: Embracing BI Tools for Accurate and Reliable Machine Learning Models
Data transformation is not often heralded for its unsung heroism in this world of machine learning; it is that foundation on which very accurate and reliable models are built. Ultimately, using BI tools in the preparation, transformation, and feature engineering of input data, a data scientist can improve the performance of their machine learning algorithms with more impactful predictions and insights to stakeholders.
For aspiring and working data scientists aiming to perfect the science of BI-driven data transformation, a good view of the best data science courses in Chennai will supersede. The programs are engineered to provide all the minute details when it concerns the theory, practicality, and sectors in using the BI tools for feature engineering and data cleaning features of machine learning projects, thus protecting students with the kind of knowledge, tools, and mindset that will be required to set up an effective machine learning solution.
As machine learning's future unfolds, the ability to harness BI tools fully and correctly will only become more critical in driving innovation, optimizing operations, and hence delivering value in an increasingly competitive and highly data-driven world. By embracing the principles and best practices of BI-powered data transformation, the vista for both accuracy and performance in machine learning models will create new frontiers for data scientists. Endeavors in the field of machine learning through data will therefore be boosted, with an eye toward progress across all industries.
About the Creator
jinesh vora
Passionate Content Writer & Technology Enthusiast. Currently Working in BIA as a Digital Marketer.
Enjoyed the story? Support the Creator.
Subscribe for free to receive all their stories in your feed. You could also pledge your support or give them a one-off tip, letting them know you appreciate their work.
Comments
There are no comments for this story
Be the first to respond and start the conversation.