AI in Pharma: Pioneering the Future of Drug Development
Innovative Solutions
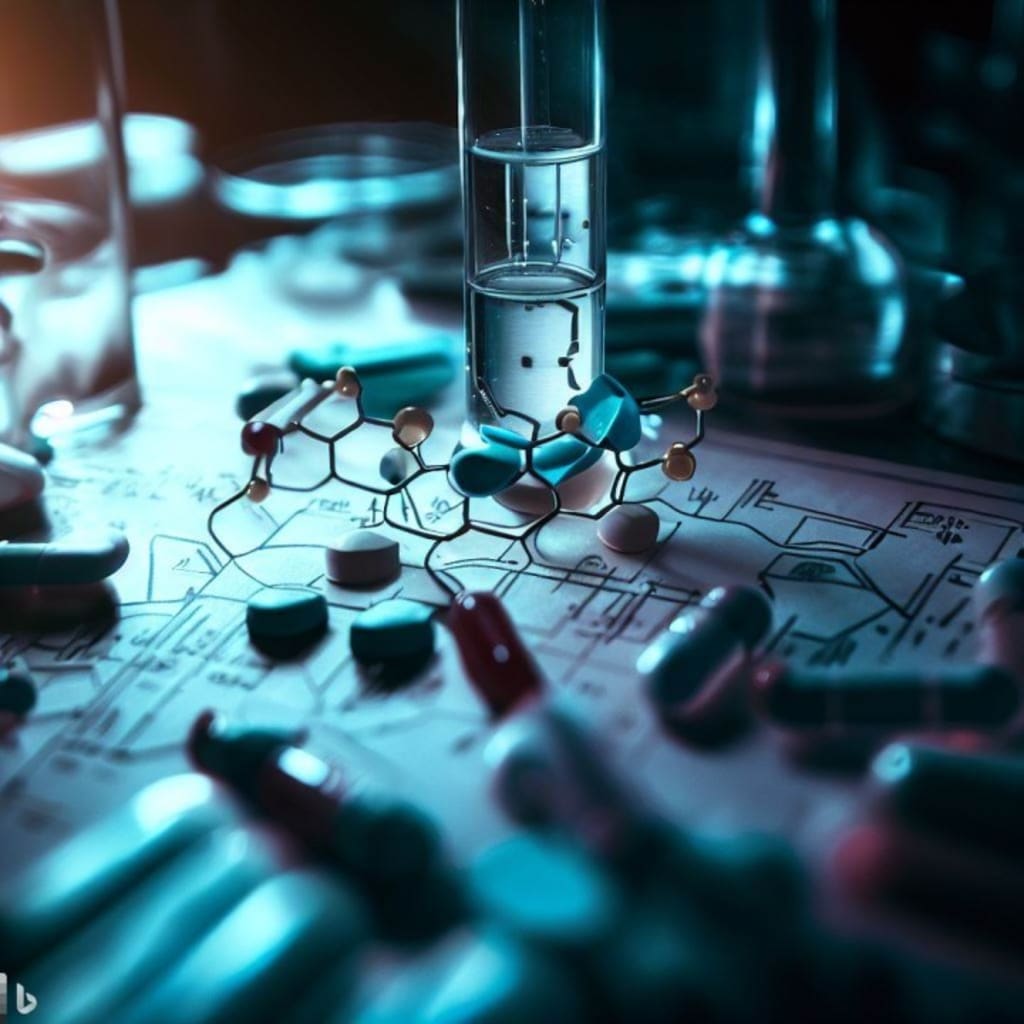
Drug development is traditionally a costly and time-consuming endeavor. However, the advent of Artificial Intelligence (AI) is poised to significantly accelerate this process, opening new possibilities for the pharmaceutical industry.
The Drug Development Process
The journey of drug development begins with identifying and validating a target, typically a biological molecule such as a gene or protein that a drug can bind to effectively. Most targets are proteins with specific sites where drugs can dock and function, known as druggable proteins.
In the discovery phase, scientists feed the target protein sequence into a computer, which then scans a vast library of small molecules to find the best-fitting drug. This approach assumes that the structures of both the target protein and potential drugs are known; otherwise, computer models are used to identify binding sites. This computational process bypasses time-consuming and costly laboratory experiments, which often have high failure rates. After identifying suitable protein targets and their corresponding drugs, the research progresses to the pre-clinical phase. Here, potential drug candidates are tested for safety and toxicity using cells and animal models. Next, during the clinical phase, the drug undergoes testing on a small group of human patients, followed by larger patient groups to assess efficacy and safety. Finally, the drug faces regulatory approval, marketing, and post-market surveillance. The high failure rate in the discovery phase limits the number of drugs advancing to subsequent stages.
AI's Role in Enhancing Drug Discovery
AI can transform target discovery and drug-target interaction analysis by significantly reducing time, enhancing prediction accuracy, and cutting costs. Two AI-based prediction tools, AlphaFold and RoseTTAFold, developed by DeepMind (a Google company) and the University of Washington, respectively, have made significant strides in computational drug development over the past four years. These tools utilize deep neural networks, which analyze vast amounts of input data to predict three-dimensional protein structures.
Recently, upgraded versions of these tools, AlphaFold 3 (developed by Isomorphic Labs, a DeepMind spinoff) and RoseTTAFold All-Atom, have advanced their capabilities further. Unlike their predecessors, these versions can predict not only static protein structures and protein-protein interactions but also interactions involving proteins, DNA, RNA, small molecules, and ions. Additionally, they use generative diffusion-based architectures to predict structural complexes. In tests involving 400 interactions between targets and small molecule drugs, AlphaFold 3 achieved an impressive 76% accuracy, compared to RoseTTAFold All-Atom's 40%.
Challenges and Limitations
Despite their potential, AI tools in drug development have limitations. They offer up to 80% accuracy in predicting interactions, with significantly lower accuracy for protein-RNA interactions. Furthermore, AI tools currently assist only in the target discovery and drug-target interaction phase; they cannot replace the need for rigorous pre-clinical and clinical testing. Another challenge is model hallucinations in diffusion-based architectures, where insufficient training data leads to incorrect predictions. Moreover, unlike previous versions, DeepMind has not released the code for AlphaFold 3, restricting independent verification and widespread use.
India's Position in AI-Driven Drug Development
Developing AI tools for drug development requires substantial computing infrastructure, particularly fast Graphics Processing Units (GPUs) to handle complex tasks. GPU chips are expensive and rapidly become obsolete. India faces challenges in this area due to limited large-scale computing resources and a shortage of skilled AI scientists, unlike the U.S. and China. Despite a rich history in structural biology fields like protein X-ray crystallography and modeling, India has not yet established a leading position in developing AI tools for drug development. However, with a growing pharmaceutical industry, India has the potential to apply AI tools effectively in target discovery, identification, and drug testing.
While AI presents promising advancements in drug development, there are significant challenges to overcome. India, with its burgeoning pharmaceutical sector, can leverage AI to enhance its drug discovery and development processes, potentially becoming a leader in this innovative field.
About the Creator
shanmuga priya
I am passionate about writing.
Comments (1)
Keep giving and present what's new now