Content warning
This story may contain sensitive material or discuss topics that some readers may find distressing. Reader discretion is advised. The views and opinions expressed in this story are those of the author and do not necessarily reflect the official policy or position of Vocal.
Save Off Strategies for Safe Design Updates
Safeguarding AI Design Updates: A Comprehensive Approach
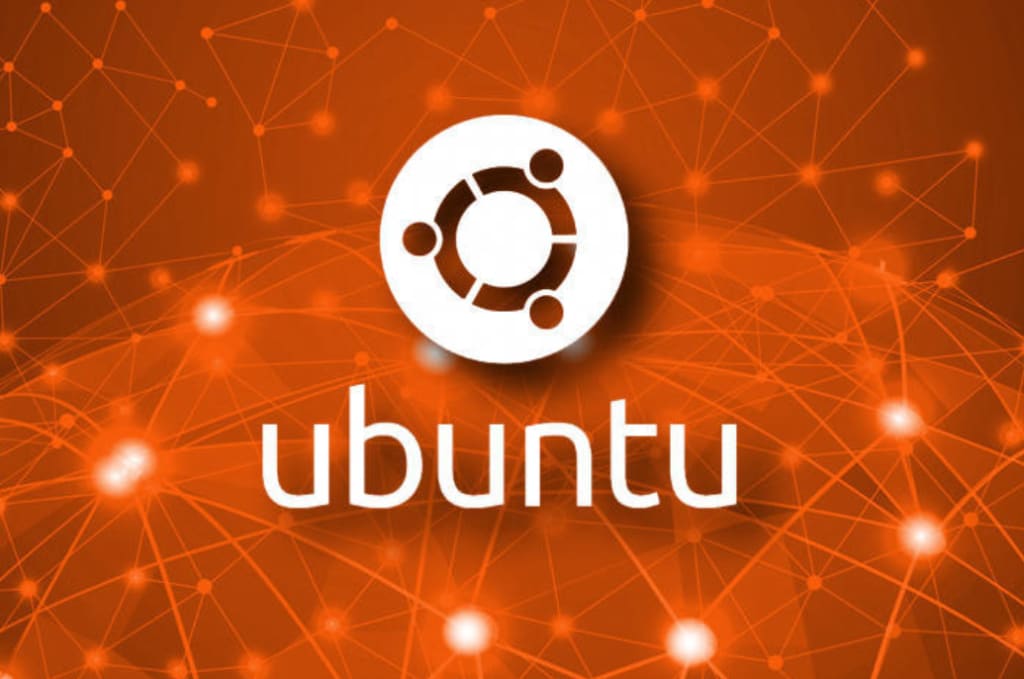
Save Off Strategies for Safe Design Updates
Safeguarding AI Design Updates: A Comprehensive Approach
As artificial intelligence (AI) continues to evolve, its integration into various sectors has become increasingly critical. Save off Design updates From healthcare to finance, AI-driven solutions promise to enhance efficiency, accuracy, and innovation. However, as AI systems become more sophisticated, ensuring their safety and reliability becomes paramount. This article explores the importance of safeguarding AI design updates, the challenges involved, and the strategies to mitigate risks.
The Importance of Safeguarding AI Design Updates
AI systems are often subject to continuous updates to improve performance, address bugs, and adapt to new requirements. These updates, while necessary, can introduce new vulnerabilities or exacerbate existing ones. Safeguarding AI design updates is crucial for several reasons:
Maintaining Trust: Users and stakeholders must have confidence that AI systems will perform reliably and ethically. Any lapse in security can erode trust and hinder adoption.
Preventing Malfunctions: Flawed updates can lead to system malfunctions, causing significant disruptions, especially in critical applications such as medical diagnosis or autonomous driving.
Ensuring Compliance: Regulatory bodies are increasingly scrutinizing AI systems. Ensuring that updates comply with legal and ethical standards is essential to avoid penalties and reputational damage.
Protecting Against Cyber Threats: AI systems can be targeted by cyber-attacks aiming to manipulate their behavior or steal sensitive data. Robust update protocols help mitigate these risks.
Challenges in Safeguarding AI Design Updates
Despite the clear need for safeguarding AI design updates, several challenges complicate this process:
Complexity of AI Systems: AI systems, particularly those based on machine learning and deep learning, are inherently complex. Their decision-making processes can be opaque, making it difficult to predict how updates will impact overall behavior.
Rapid Evolution: The field of AI is rapidly evolving, with new techniques and models emerging frequently. Keeping up with these advancements while ensuring safety and reliability is a continuous challenge.
Data Sensitivity: AI systems often rely on vast amounts of data, some of which may be sensitive or personal. Ensuring that updates do not inadvertently expose or misuse this data is critical.
Interdisciplinary Nature: Safeguarding AI updates requires expertise from multiple disciplines, including computer science, cybersecurity, ethics, and law. Coordinating these diverse perspectives can be challenging.
Strategies for Safeguarding AI Design Updates
To address these challenges, a comprehensive approach to safeguarding AI design updates is essential. This approach should include technical, organizational, and regulatory measures:
Technical Measures
Robust Testing and Validation: Before deploying updates, thorough testing and validation processes should be in place. This includes unit testing, integration testing, and system-level testing to ensure that updates do not introduce new issues.
Version Control and Rollback Mechanisms: Implementing version control systems allows developers to track changes and revert to previous versions if an update causes problems. Rollback mechanisms are particularly important for quickly addressing critical issues.
Automated Monitoring and Anomaly Detection: Continuous monitoring of AI systems can help detect anomalies or unexpected behavior following an update. Automated tools can flag potential issues for further investigation.
Adversarial Testing: Simulating potential attacks or adversarial scenarios can help identify vulnerabilities that may not be apparent during regular testing. This proactive approach can strengthen the system's resilience against malicious activities.
Organizational Measures
Cross-Disciplinary Collaboration: Fostering collaboration between AI developers, cybersecurity experts, ethicists, and legal professionals ensures that all aspects of AI safety are considered during the update process.
Transparent Communication: Clear communication about the nature and purpose of updates helps build trust with users and stakeholders. Providing detailed release notes and engaging with the community can enhance transparency.
Continuous Training and Education: Keeping the development team informed about the latest best practices in AI safety and security is essential. Regular training sessions and workshops can help maintain a high level of expertise.
Regulatory and Ethical Measures
Adherence to Standards and Guidelines: Following established standards and guidelines, such as those from the IEEE or ISO, can provide a solid foundation for safe AI updates. These standards often incorporate best practices and regulatory requirements.
Ethical Considerations: Ethical considerations should be integrated into the update process. This includes evaluating the potential impact of updates on fairness, privacy, and bias. Engaging with ethicists can help navigate these complex issues.
Regulatory Compliance: Ensuring compliance with relevant regulations, such as GDPR for data protection, is crucial. This may involve conducting regular audits and assessments to verify that updates meet legal requirements.
Case Studies and Best Practices
Examining real-world case studies can provide valuable insights into effective strategies for safeguarding AI design updates. For example, Design updates the healthcare industry has implemented rigorous validation processes for AI-based diagnostic tools to ensure patient safety. In the automotive sector, companies developing autonomous vehicles conduct extensive testing in simulated environments before deploying updates to their fleets.
Best practices from these industries can be adapted and applied to other domains. Key lessons include the importance of a multidisciplinary approach, the need for robust testing frameworks, and the value of transparent communication with stakeholders.
Conclusion
Safeguarding AI design updates is a multifaceted challenge that requires a holistic approach. By combining technical, organizational, and regulatory measures, it is possible to mitigate risks and ensure the safe and reliable evolution of AI systems. As AI continues to advance, maintaining a strong focus on safety and security will be essential for building trust and unlocking the full potential of this transformative technology.
About the Creator
Ratul Munshi
I am An Seo Expart
Comments
There are no comments for this story
Be the first to respond and start the conversation.